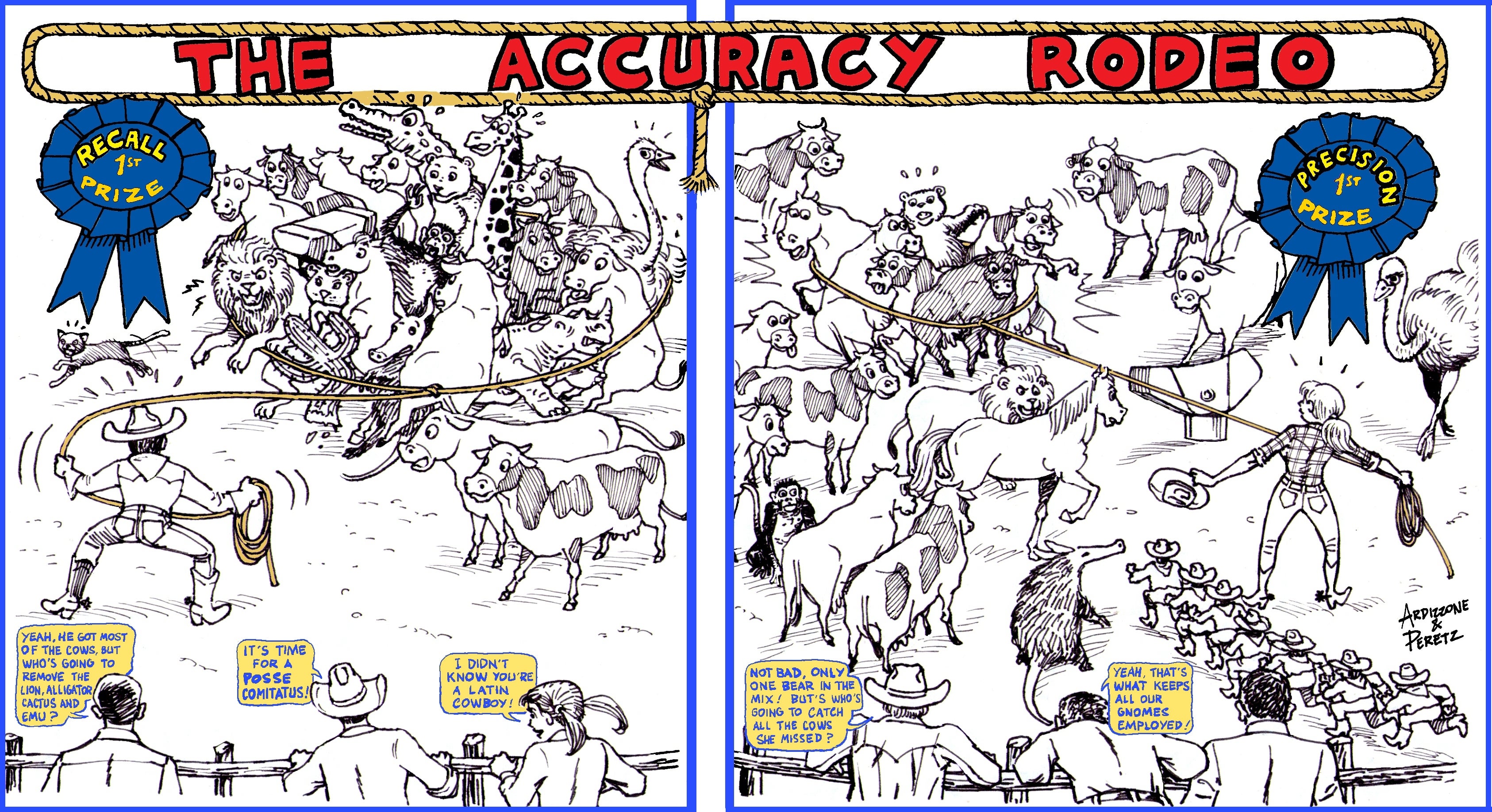
Your company has tens or hundreds of thousands of agreements, most of which pre-date your tenure or you inherited from acquisitions. Who has time to re-read them all to figure out what’s in them?
Is artificial intelligence (AI) the answer? If you believe the occasional press releases touting AI tools, all you need are to load a few sample contracts, provide a few minutes of training input, and then the computer will read all your agreements for you.
This scenario is as likely in the present day as your smartphone suddenly functioning as a tricorder from Star Trek with the mere download of a new app. It’s true that your phone, when combined with some add-on gadgets like a breathalyzer, might someday be able to identify specific limited conditions. However, we are far from eliminating doctors in the field of medical diagnosis.
Similarly, we are not yet at the stage of eliminating lawyer oversight of artificial intelligence systems tasked with reading your agreements. The goal of this article is to explain how to judge the efficacy of AI tools that may be applied to your legal documents.
A recent Quislex study examined 10 AI tools that claimed to abstract key terms from business agreements. Of the vendors surveyed, only half met enterprise-level core requirements for security, integrations, batching, and searching.
Five of the vendors were tested on 48 typical commercial agreements, including servicing agreements, license agreements, purchase orders with terms of service, and supply agreements. The result was a time savings of between 16 percent and 36 percent across the five systems, with an average of 28 percent in time savings.
Each of these AI tools is comprised of a series of models. There is typically a separate model for each key term (e.g., governing law, effective date, confidentiality requirement) that the tool is hunting for in a contract.
Tests of the accuracy of these tools revealed that some of their models were far better than others. Specifically, the strong suit of four of the five tools tested was noting the absence of certain basic clauses, which these tools performed at a greater than 85 percent success rate.
Unfortunately, when the same tools were asked to find clauses that were present in the agreements, they often erred. The tools achieved their highest success rate (78 percent) identifying governing law. However, they were correct on average less than 50 percent of the time trying to identify notice provisions, limitations of liability, and insurance requirements.
What does this study of AI tools bode for identifying the key terms in your own company’s agreements? First, it’s unlikely that the tools can run unsupervised because your internal clients are not going to be satisfied with you finding only half of the insurance requirements that bind your company. Thus, you need to be prepared for a major legal quality control investment to achieve the standards that your colleagues expect from the legal department.
Second, the productivity impact of these AI tools is material, but not enough to obviate the need for lots of lawyers to operate, train, and manage the tools. Imagine that an excellent lawyer could read a typical agreement in 90 minutes and identify and extract all the business terms. If you have 10,000 agreements, that is 15,000 hours of lawyer time.
Even with the average 28 percent time savings that Quislex found from AI tools, you would still need 10,800 hours of lawyer time to get through all those agreements. With the average lawyer handling 2,000 hours of work per year, that represents five full-time lawyers for a year.
The arch rivalry between precision and recall
Let’s imagine you do have enough spare lawyers to train, operate, and quality check the output of AI tools. What are the criteria to apply when assessing which AI tool to use?
Whether you are searching for on-point caselaw or identifying and extracting key terms from a contract, as a lawyer you typically have two competing goals:
- Find everything you are seeking and don’t miss anything.
- Don’t waste time culling through incorrect or inappropriate responses.
If your net is not cast widely enough, you may miss clauses or documents or cases that you really wanted to find. On the other hand, if your net is cast too widely or incorrectly, you will receive scores of inappropriate results that waste days or weeks of your time to cull through.
In AI terms, these competing goals of being widely inclusive while still avoiding false positives are described as “recall” and “precision.” To save time for its human operators, AI models need to be high in both precision and recall. A model high in only one of those metrics will result in either unnecessary work or incorrect results that are worse than having applied no AI tools at all.
Precision is a measure of how often you have incorrect answers. For example, if you are supposed to identify all the parties to an agreement, you would have high precision if you did not accidentally designate a non-party as a party.
High precision alone, however, is not a sufficient recipe for success: An AI model with high precision may present you very few false alarms. However, it could also miss a lot of correct answers due to its conservatism and bias toward avoiding any answers that might possibly be incorrect. A highly precise model is like a person who is so afraid to get an answer wrong that they refuse to take on challenging problems.
By contrast, recall measures whether you cast the widest net necessary to catch all possible instances of what you are seeking. Imagine you are hunting for all references in a document to exclusivity. A model with high recall might catch all such references, but also flag lots of other sections that don’t refer to exclusivity, for example.
The result is that a model with high recall, but poor precision, which could have lots of false alarms that require a human to investigate. A high recall model is like a person who subscribes to every newspaper and magazine in the country, so she doesn’t miss an important story, with the result being that she has endless chaff to sort out in order to find the wheat.
Understanding the concepts of precision and recall are essential for assessing AI tools because shortcomings in either one — or an imbalance between the two — can result in the need for lots of lawyer time to be spent. If someone claims that their AI tool is accurate, make sure to get data on both its precision and recall, because a high score in one without the other will lead to a lot of extra work for you in eliminating false positives or finding the missed answers.
And, as demonstrated by the Quislex study, each AI tool is comprised of a series of models, with one model for each clause or term you are seeking to find in a contract. Bear in mind that precision and recall likely vary for each of these models, which means that an AI tool with fantastic recall or precision for one type of clause (e.g., governing law), may be far less accurate when you want to identify agreements containing other clauses (e.g., a change of control trigger).
Your time is valuable and outside lawyers are expensive. Thus, you need to choose your AI tools carefully or else they could be a major time sink for you or big expense for outside lawyers to train, manage, and quality control. When evaluating any AI tools, ask the following:
1. What is the precision and recall of each model provided by the tool?
A lopsided ratio of precision to recall foreshadows the extent to which you will be spending your time eliminating false positives or hunting for missing answers, or perhaps both if precision and recall are equally lacking. A model with a high score in just precision or just recall may end up requiring more time to correct than starting from scratch.
2. How many AI models does the tool have?
Each piece of information you are seeking will likely require its own model and training and have its own precision and recall score.
3. What was the heterogeneity of the data used to train the AI tool?
AI tools that have not been trained on a wide variety of agreements are likely to have misleading precision and recall scores because they are optimized for an artificial environment that is not representative of the real world. By analogy, it’s easy to become good at identifying each type of fish in the pet store, but that won’t prepare you well to name all the fish in the Indian Ocean.
4. What is the output format of the tool?
You need a tool where the results can be quality reviewed by your team of lawyers and compared to the source documents.
5. What systems does it connect to?
Any system that finds data for you will not live up to its potential if you cannot feed the resulting output to all the business stakeholders in your organization. For example, if you are analyzing client agreements, you will want to be able to push data to popular customer relationship management systems like Salesforce.
6. How granular are the system’s results?
There is a big difference between finding a certain section of an agreement versus interpreting the specific terms in that section. For example, an AI tool that directs me to an assignability clause still requires me to read that clause and determine the conditions under which my agreement is freely assignable. By contrast, an AI tool that specifically identifies which of my business agreements are freely assignable saves me far more time.
7. What role did lawyers play in developing and improving the tool?
Many software tools and AI systems are developed by individuals with a software and data science background, not a legal background. Look at the senior team and staffing of the company making the AI tool to verify that they have experienced lawyers onboard and on the senior team providing a direct feedback loop to the software developers. Otherwise, you might end up with a tool that makes sense to an engineer but not to the practicing lawyers who need to operate it.
8. Are there enough lawyers on staff to train the tool and review the results?
Find out from other users how much lawyer time was required to train the tools for their application and verify the results. When evaluating case studies, consider whether the contracts reviewed were as varied as yours.
Claims in press releases are not a substitute for hard data. Understanding the independent concepts of precision and recall and the fact that the strength of each AI tool can vary — depending on which term it is seeking in your agreements — will make you a savvier evaluator of options.