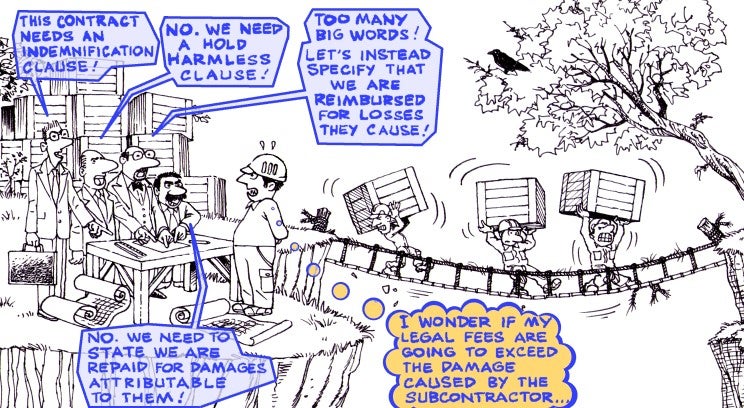
On a regular basis, we are presented with headlines explaining how artificial intelligence (AI) is going take over our work so we can glide around on self-driving electric scooters all day while being hand-fed by robots. Yet when we go home and ask Siri to turn on the “lights,” it instead offers us treatment for “lice.”
It’s quite easy to test out Siri and Alexa ourselves — or watch funny online videos of their failure, but how should we go about assessing the potential for AI to help us with legal work, such as interpreting contracts?
In this article, we will give you a framework for understanding some of the challenges in applying artificial intelligence to understanding legal contracts. Once you understand these challenges, you can better assess the likelihood of such technology improving aspects of your work life.
What is all this talk about training?
You spent years training your dog to fetch sticks and perhaps even longer training junior associates to spot hidden risks in insurance contracts. But why do we need to train the artificial intelligence?
When reviewing and drafting contracts, there are many ways to describe a particular business term or concept. Take something as simple as “effective date.”
How many ways could you say that? Rather than using the word “effective,” an agreement might instead refer to the date of implementation, kick-off, start, initiation, beginning, commencement, activation, opening, launching, embarkation, or bust a move.
Of course, each of those words could vary as well: Is it the date of kick-off, kick-off date, time of kick-off, or when we kicked off?
The concept of an effective date has a finite number of variations, yet we still cannot develop a list in one sitting that encompasses all the ways it could be described. The same applies to many other legal concepts, such as indemnification. Some attorneys prefer the terminology “hold harmless” while others refer to it as a “make whole” clause. Even more variations are likely.
Rather than trying to catalog all the words that could describe a legal concept, such as effective date or expiration date, and the many potential conjugations and tenses of each of those words, machine learning is “trained” to find words by observing how experts have handled similar situations.
If a concept or business term can be described in just a few ways, then training is more limited. If a business concept can be described in many different ways, much more training is needed.
Seek out heterogeneity
Consider the concept of an expiration date. How many ways could that concept be described? Lots. There are so many more permutations possible for describing an expiration date, some of which also require a reference to other terms (intermediate variables) in the agreement.
For example, an agreement could expire or auto-renew on the third anniversary of the second month after maturity date. And the notice date might be 23 days before the expiration date. In order to train a machine to decipher the expiration date, we need to train it to understand that an intermediate variable is involved and how to find it.
Proper training of machine learning models requires scores of heterogeneous examples. When training a machine to find all the variations of a business term, it is essential to not rely on scores of standard form agreements where only a few variables, such as date or price, are changed from one document to the next.
These standard forms are essentially thousands of copies of the same agreement, with only a minor variation. Standard forms lack the heterogeneity to train machines.
Only experts need apply
Human attorneys have a base of knowledge prior to reviewing a contract that helps them generalize from description to others. This is called transfer learning.
Lawyers have spent at least 19 years in school before reviewing their first contract as a licensed attorney. These decades of prior education mean that even the newest attorney has already seen countless variations of how a concept could be described.
By contrast, machines lack transfer learning, so they need extensive training on hundreds or thousands of variations of a contractual term as possible before they can be relied upon to identify it themselves.
When training machines to understand technical documents, like contracts, it is essential that there are true subject matter experts in the loop. You may have seen “captchas” on the internet, which seek to determine who is a human and who is a robot.
The tasks that the humans are asked to complete are relatively simple, such as identifying which images represent a donut and which image is part of a street sign. Even uneducated humans are likely to be able to complete such a task and do so consistently in the same way that other humans would judge the situation.
By contrast, when dealing with legal documents, such as contracts, we cannot simply rely on any breathing human to provide appropriate supervision and training. Far more people can recognize a street sign than can determine whether an indemnification is mutual or one way.
So, we just make sure the human trainers have a law degree, right? That’s a start, but it is still insufficient for training a model because attorneys often disagree. You cannot build a solid machine learning model if the attorneys are not in agreement.
If human experts cannot agree, the machines cannot do so either. In order to build solid computer models, the developers of the models need to impose rigor and discipline upon the human experts who are training and tuning the models.
This process is called inter-annotator agreement. To reach inter-annotator agreement, the model builders must examine the human experts and understand where they disagree with each other and then have other human experts involved to break the tie.
Invest in consensus-building
Tightly managing the human experts for training a model is akin to how the most rigorous bar exams are graded. For the California Bar Exam, for example, graders are selected from those who scored highly on their own exam. Groups of 12 graders meet three times. In each session, the graders discuss sample answers and the criteria for determining the sufficiency of each one.
They use this process to see if they can reach consensus on subsequent answers. They must re-read answers where they disagree and try again to reach consensus. In certain circumstances, such as exams below a certain score, if the difference between two graders is more than 10 points, then a supervisory grader is brought in as well.
This same type of rigor needs to be applied to training models to review agreements: The human experts need to reach consensus before enough data is accumulated to train a machine for the task.
The reason that bar associations make such an investment in calibrating graders is that the consequences are so high — an applicant’s livelihood is at stake. A similar perspective needs to be applied to training models: The model will have an ongoing daily impact, long after the model trainers cease their involvement.
The necessity of rigorously managing human experts who train models makes it hard for most organizations to calibrate and tune models on their own. When a big training task arises, such as the review of thousands of agreements, companies lack enough internal staff to have their day-to-day team running the entire review process.
Instead, temps and outsourcing firms are hired for these seemingly one-time tasks. These individuals have not worked together before and are typically paid and incentivized based on hours worked, rather than consensus-building. There is rarely a management and quality control layer in place above these attorneys to impose sufficient rigor.
Even in your own organization, you have probably noted that attorneys and other executives regularly “agree to disagree” about what certain agreement terms mean, rather than make the investment to develop a consensus viewpoint.
For the few organizations that make the investment in building a consensus on how particular terms and concepts should be interpreted, there is a direct beneficial business impact for their business.
These organizations can start benchmarking their own documents and make apples-to-apples comparisons. They can start to compare agreements and clauses to discover the best role models to follow, as well as outliers among their agreements that need to be renegotiated. This benchmarking provides important context for subsequent transactions.
Until we have found a way to rebuild the attorney equivalent of the Tower of Babel, training AI systems will continue to be challenging. Success will be attained by those willing — and able — to rigorously quality control and manage attorney experts, train based on a wide range of heterogeneous examples, and invest heavily in assessing inter-annotator agreement and building consensus.