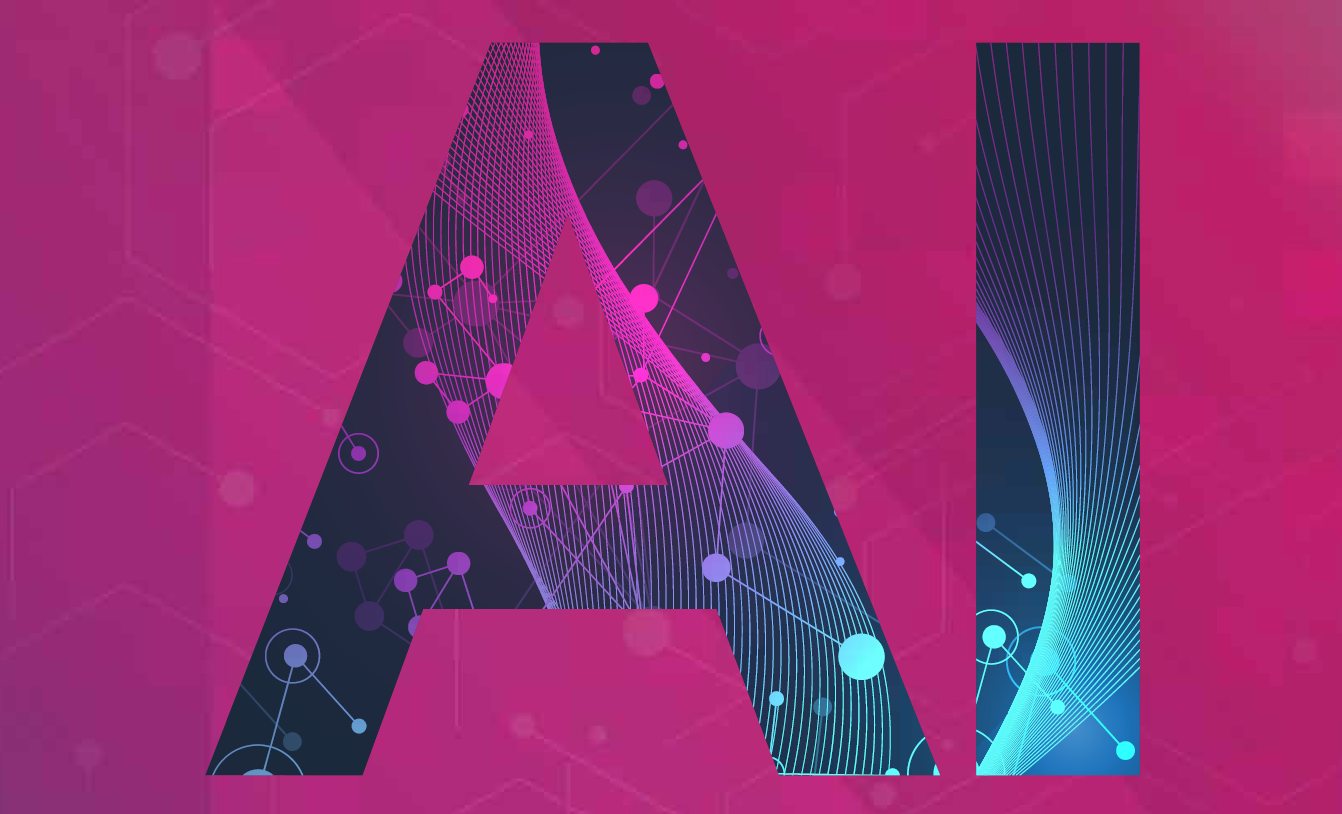
CHEAT SHEET
- Artificial intelligence. AI is fast becoming standard across sectors, including legal.
- What goes in must come out. AI is only as good as the input data, and no algorithm can exercise human discretion.
- Clarity. The ability to accurately convey and explain your results is just as important as the information itself.
- Pace of development. AI is developing faster than the laws that regulate it. Work out legal gray areas like output ownership before you seal a contract.
If your company isn’t using artificial intelligence already, it will be soon. As one major US telecom says, “Companies not actively exploring AI in their roadmap plans face being left behind.” For GCs and other in-house counsel, the evolving nature of AI raises important challenges in compliance and risk management. Some of these can be addressed on the front end with thoughtful contracts and indemnifications — if you know what to look for. Other issues need to be monitored on an ongoing basis, because what makes AI powerful makes it harder to manage: its ability to learn and change.
Questions counsel should consider include: How autonomous is the technology? What is the risk profile of the technology and the use case, for errors of both inclusion and exclusion? What are the inputs and feedbacks of the model — and who defines and categorizes them? Are there “humans in the loop” and if so, who manages them?
If your company isn’t using artificial intelligence already, it will be soon. As one major US telecom says, “Companies not actively exploring AI in their roadmap plans face being left behind.” For GCs and other in-house counsel, the evolving nature of AI raises important challenges in compliance and risk management. Some of these can be addressed on the front end, with thoughtful contracts and indemnifications — if you know what to look for. Other issues need to be monitored on an ongoing basis, because what makes AI powerful makes it harder to manage: its ability to learn and change.
This article is not about so-called general AI, the stuff of movies that is closer than we think but not here yet. General or “strong” AI involves algorithms that don’t just solve tasks but know what a task is and react with consciousness. That will present its own set of legal issues, and predictions about its arrival should be tempered with some humility. A 2015 Oxford survey of 352 experts predicted AI would surpass humans in playing the Chinese game Go in 2027. It happened in early 2016.
But even today’s technology — the neural networks that don’t feel but solve tasks at superhuman levels — presents important legal issues requiring thought and planning. Far from the domain of trendy startups, major companies are already adopting these technologies across industries. A leading US bank, noting that 80 percent of loan servicing errors were due to human mistakes in contract interpretation, adopted machine learning. It can now extract 150 legal variables from 12,000 commercial credit agreements annually, with the added benefit of cutting its yearly review time from 360,000 hours to mere seconds. In medicine, AI systems are diagnosing skin cancer and predicting adverse hospital events earlier and sometimes better than physicians. Companies across sectors are using AI to predict orders, ship cargo, spot defects, manage customer service, and detect fraud. AI is here.
So, what is a good in-house counsel to do? Consider it the wave of the future and jump in? Wait for industry norms and regulatory standards to evolve? Adopt off-the-shelf solutions or seek bespoke services? Each approach has its own risk profile, but those judgments should be informed by a working understanding of what AI is and how it is both similar to and different from past technologies.
Questions counsel should consider include: How autonomous is the technology? What is the risk profile of the technology and the use case, for errors of both inclusion and exclusion? What are the inputs and feedbacks of the model — and who defines and categorizes them? Are there “humans in the loop” and if so, who manages them? Are there regulations or guidance proposed or adopted for the sector? What does the data show about overriding the system when its recommendations are counterintuitive? What are others in the same industry doing, both in terms of adopting these technologies and in monitoring them? Are there checks in place to flag problematic outputs? How are these and other risks shared or divided in the service and product agreements?
All of these questions can affect the safety, reliability, and economics of AI, including liability risks. In a rapidly evolving environment, having answers to these questions on the front end, and revising systems as AI technologies evolve, is key. In speaking with companies on these technologies, whether making them or adopting them, the following topics come up again and again.
AI: What is it?
A wry observer once pointed out that under many definitions in use today, even a calculator would qualify as AI — it automates tasks that were once the domain of human intelligence. That has led to the tongue-in-cheek definition that AI is whatever we haven’t invented yet. Early AI technologies were often expert systems, which try to codify human thinking through decision-trees and if-then statements. That approach was fundamentally limited. It might be faster or more reliable than people, but it would never see further than the humans it was designed to emulate.
What most people mean by AI today is machine learning, algorithms that can actually evolve and write their own decision-trees in response to data. Neural networks, a familiar but rarely understood concept, are one example of machine learning, deepening that process through feedback loops and multiple layers of analysis, much like the human brain does.
But even neural nets can be demystified for lawyers. Even the most math-phobic among us JDs are familiar with correlation, the process of looking for relationships between variables. Many of us have worked with expert witnesses to see if a line can be drawn between, say, an alleged harm and the economic damages sought. Neural networks look for relationships too, but instead of simple straight lines, they use complex non-linear equations to test arbitrary relationships among a vast number of variables, over and over, in different combinations across deeper layers. The network tests against known data to prepare a hypothesis or model, then challenges that model against new cases, refining the algorithm as it goes. The same neural network could produce different models from the same data. The only question it asks is: Does it work?
On the one hand, this process can lead to predictions far beyond any simple model. On the other hand, the formulas may become so complex and flexible that they can be inscrutable to the human mind. In many technologies, that may not matter. I don’t need to know how my phone works in order to make a call. But where human judgment is intertwined and the stakes are high, relying on machine recommendations that may be counterintuitive and lack an understandable basis presents complicated legal and compliance issues.
Artificial intelligence for in-house generalists: Back to basics with advanced analytics
The legal dimensions presented by AI can seem daunting — particularly to in-house attorneys who do not specialize in technology, or who work in fast-paced corporate environments. How can in-house counsel add value to everyday discussions in this space? What else can we do besides raising data privacy, intellectual property, and other issues outlined in this article?
Those of us who are not technology focused can contribute significantly to company performance and risk mitigation by improving AI fundamentals such as inputs and testing. While technologies evolve continuously, a core competency for in-house counsel remains constant: knowing as much as possible about our businesses to support compliant, smart risk-taking.
This translates well to minimizing “garbage in, garbage out” in the AI context. We can leverage our operational expertise to identify, collect, and clean the data that feeds our AI, and to refine its training parameters. For example, through our seats on governance boards, the contracts we negotiate, and the myriad queries we triage from stakeholders who may not have visibility to each other’s projects, in-house lawyers hold substantial institutional knowledge on what information we have and where.
This is a tremendous asset when relevant data is often siloed geographically, within departments, or otherwise. Whether we advise broad constituents, such as entire regions or business units, or provide more specialized support, in-house attorneys can help scope and connect dispersed data. Achieving this initial step of locating, collating, and refining pertinent internal data — including ensuring uniformity in formatting and definition (what certain labels or nomenclature mean, or should mean) — can be a major win itself, especially in large, matrixed companies. We first need to have a good handle on the information we already possess to determine next steps, such as additional data we should acquire externally to fuel our analytics-driven strategies, deploying AI or otherwise.
The need to “clean” data, collect more as needed, and train AI allows lawyers to capitalize on other core competencies: precision in definitions and pressure testing. Our deep understanding of the enterprises we advise — coupled with our ability to see multiple plausible interpretations, clarify terms, play devil’s advocate, pose hypotheticals, and spot potential biases — can make AI substantially more accurate. In terms of mitigating risk, there may be no better measure than getting the right outcomes, even if — or particularly if — it is not always clear where liability, if any, resides when it comes to AI.
In short, we can help make AI less artificial and more intelligent by reverting to the basics behind good analytics — quality inputs and testing — that our daily jobs and training as attorneys equip us well to provide.
“Garbage in, garbage out”
An algorithm is only as good as its inputs. Counsel whose companies are adopting AI should work to understand the nature of the data used to train the system, as well as the nature of the data used to run the system once it is trained. The adage “garbage in, garbage out” applies to AI in spades. The best system trained on poor data will produce poor results, even if your company runs the system on excellent data. Even if an AI is trained on good data, if there is a mismatch between that data set and yours, unexpected results may occur.
Locked or continuous?
Apart from the data, not all AI is created equal. Especially in hype cycles where companies face pressure to adopt new technologies, it is sometimes lost that not every solution-promising machine learning is as good as another. Perfect data cannot save a poorly designed and implemented algorithm. That is one of the considerations behind the FDA’s new test approach to AI regulation, the Software Pre-Cert Pilot Program, which looks to the quality of the company and its processes rather than, in the first instance, at the product. As the FDA’s Digital Health team notes, this is a departure from the way traditional medical devices are regulated, but it reflects the challenges of regulating AI.
AI algorithms can change. That is their strength and their weakness. General counsel should understand whether AI solutions are locked or continuously learning. Locked systems are trained and then frozen in time. From a quality perspective, that makes them more like traditional tools where quality is assessed mainly on the front end — although one risk of locked systems is that local user data can drift away from training sets over time. A tool that is good for time X may not be right for time Y. On the flip side, continuously learning systems have their own strengths and weaknesses. They can evolve to meet new challenges and conditions, but they can also shift or drift in the wrong direction, so issues like version rollbacks and logs need to be considered. AIs, like people, can move in the wrong direction and need help to find their way back.
The AI made me do it
AI raises unique challenges for determining causation. AI has touchpoints between people and machines at many steps along the way, inside and outside a company. Recent cases have pointed out that it’s hard enough to determine causation in the massive code of traditional software. When an AI system goes wrong, with an inscrutable algorithm and various human-machine touchpoints across the life cycle, how does one begin to assess fault? Absent statutory or contractual solutions on the front end, untangling such disputes will involve the common law process. Limitations of liability and indemnification provisions can provide clarity and predictability, but these provisions must be carefully crafted to address the carveouts and limiting conditions that can render them unhelpful.
Moreover, in regulated professions, reasonable reliance is a key issue. When decision-assist software produces unexpected or inappropriate recommendations, stakeholders within a company will seek guidance from counsel on how to respond. Traditionally, legal theories like the “informed intermediary” or “learned professional” doctrines can limit a highly trained worker from claiming rote reliance on machines as a legal shield. As the accuracy of these systems continues to surpass that of human operators, that doctrine will face pressure. For example, a judge’s decision to rely on bail-setting software to release a defendant led to dire results. As one district attorney then noted, it is very hard for professionals to ignore recommendations couched in science. And yet, in the end, it was a human data-entry error that ultimately led to the bad machine recommendation.
General counsel should also be aware of the AI concepts of transparency and explainability. If an AI system can see farther faster, can it at least explain back to human operators how it got there? Some imagine a technical tradeoff between precision and explainability — the more an AI is shackled by having to explain its reasoning to people, the less robust its predictions will be. In such cases, counsel will need to weigh the value of oversight against the value of efficacy and set the appropriate tradeoffs.
General counsel should understand whether AI solutions are locked or continuously learning. Locked systems are trained and then frozen in time.
Avoiding bias
Algorithmic bias is a pressing and real issue. Reliance on facially neutral algorithms can still produce outcomes that are discriminatory, and that discrimination can range from unfair to unsafe. Safety sensors trained only on one demographic can literally fail to recognize and protect people who don’t look like the training data. In lending, hiring, leasing, policing, and beyond, input data that reflects societal biases can produce biased algorithms and outcomes that invite legal challenge. These errors can be inclusionary or exclusionary: leaving people out unfairly or lumping them in unfairly. And even if impermissible attributes are coded out of the software, the organic evolution of proxy variables may lead to the same improper conclusions. Counsel should investigate how particular AI solutions handle these issues, from ensuring that input data is diverse to weeding out proxies and testing outcomes for improper bias.
Innovate or die?
Pace of adoption is a matter of risk balancing as well as business pressures. Waiting for industry and legal standards to evolve is one approach. But waiting for the law to evolve may leave a company open to serious risk should a legal problem arise. That is, there are costs to consider on both ends of the adoption curve.
You don’t know me! Or do you?
Companies and counsel should pay careful attention to privacy and IP issues. How do you protect customer information when AIs can readily deduce people’s secrets and invade their privacy? One big box retailer learned this the hard way when its algorithms deduced a pregnancy based on fluctuations in cotton and lotion purchases — sending an automated maternity coupon to the customer’s father. Already, numerous statutes are defining new areas of privacy that counsel should ensure their AI systems will consider. And on the IP side, questions of who owns the output of AI should be negotiated in advance to avoid current legal gray areas.
These are some of the issues to consider and manage when adopting AI systems. This is a tremendous new technology that will lead to better results for many people. But it will present new challenges and concepts for in-house counsel. Working to create good AI will be a team effort. Counsel need to understand what AI is and how its many variables can affect safety, efficacy, and quality. Just as watch companies and car companies never thought they’d become software companies, lawyers are now living in a rapidly changing digital era that will transform their everyday practice.