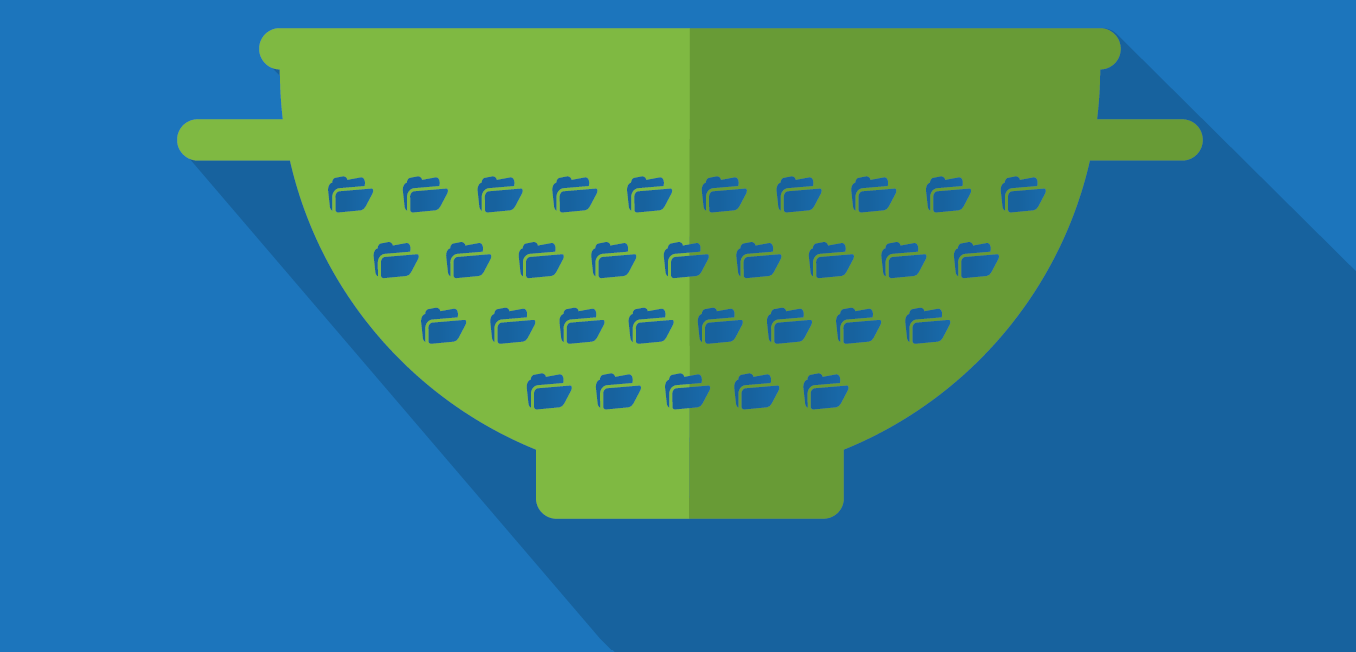
CHEAT SHEET
- Learning to pry. Human feedback into the review process enables an algorithm to “learn” to identify relevant documents.
- Louder than words. Sentiment analysis identifies patterns of language to determine the emotion associated with it, as a grouping strategy.
- Welcome to the machine. Given that legal analytics is an emerging field, firms and legal departments may need to hire data scientists or outside consultants to implement this technology.
- Us and them. Social network analysis enables investigators to visualize communication patterns of interlinked individuals by frequency and topic.
Almost 600 years ago, Johannes Gutenberg’s printing press profoundly changed how people managed and used information by applying the principle of mass production to data. The digital revolution, which began mere decades ago, has amplified that transformation in almost inconceivable ways. Today, the universe of recorded information globally is mostly digital. It’s enormous, expanding, and evolving. In fact, many people today find themselves trying to stay afloat in an ocean of “information overload.”
“Information Overload” entered our vernacular through Alvin (and Heidi) Toffler’s prophetic book Future Shock.
The historic approach to understanding data was to read it, much like the books from Gutenberg’s first printing press were read. But with the extreme volume of data that has been created and stored since the digital revolution began, the real challenge today is simply having the bandwidth to read everything that has to be read, particularly in knowledge service industries, where understanding all relevant facts is essential. This couldn’t be truer than in the litigation discovery context. In an investigation or litigation, all of a company’s Electronically Stored Information (ESI) is potential evidence and potentially “discoverable.” This phase of litigation is known as “discovery” and has more recently been recast as “e-discovery.” Regardless of the label, attorneys involved in an investigation or litigation discovery still have to determine what is relevant, what is protected from disclosure, and what is important to the theory of a case. Now, they have to do so while managing the volume, variety, and velocity of ESI. Meeting this basic age-old challenge in a digital world has led to the development of new data analytics tools, including advanced text analytics (ATA), which are changing the way vast amounts of data are now “read” in litigation and investigations.
The threshold concern is how professionals can “read” and synthesize the enormous and growing volume and variety of data for which they are responsible. Can new analytics technologies help ease the process and make it faster? Can it help professionals better identify what is important, what must be turned over in discovery, and what must be shielded from disclosure? The answer to all of these questions is a qualified “yes.” Technology is merely a tool, and as with any tool, the quality of the outcome is governed more by skillful use than the tool itself.
At a fundamental level, analytics tools are used to facilitate insight by 1) organizing data in specifically useful ways, and 2) presenting them in a manner that fosters insight. In legal discovery, the focus is on text and document metadata, so most tools use unsupervised or supervised learning techniques to identify and group documents according to text patterns. This definition is as abstract as the technologies and processes themselves, but this article aims to clarify the important concepts below, because these techniques, especially ATA, are changing the productivity paradigm for lawyers who have to read enormous volumes of electronic documents to identify potential evidence.
The legal document review process has fundamentally different economics and logistics when ATA tools are involved. Data volumes and money spent on e-discovery are growing rapidly, but how the work gets done and who is doing it have the most dramatic impacts on costs and outcomes. Companies, law firms, and document review firms that develop expertise with these new tools and approaches will be winners. Those who fail to adapt to the new environment will be left behind. Embracing these new tools also will be essential for any company that wants to limit its total legal costs in the future.
For corporate counsel to take advantage of these new technologies, they must understand what tools are available and what purposes they serve best. Major available and emergent techniques are described below. Cost and accuracy are the most important criteria for evaluating the utility of “analytics” in discovery, and document review is still the largest component of e-discovery spending.* As a result, the greatest savings in litigation spend use analytics strategies to lower costs by reducing the number of documents to review and/or increasing the speed and consistency of review.
* NN Pace and L Zakaras, Where the Money Goes - Understanding Litigant Expenditures for Producing Electronic Discovery; RAND Institute for Civil Justice, 2012.
Lawyers today know that reading all the ESI is simply not practical because of limited budgets, the increased use by courts of so-called “rocket dockets,” and the high prevalence of irrelevant data. Those unfamiliar with the newer ATA tools use limited but well-known techniques like Boolean search to limit the amount of review required, as well as cheaper, outsourced reviewers to lower costs. They also try to force-fit familiar data review approaches to meet all of their needs, even though other available solutions would work better from both a cost and effectiveness standpoint. For example, to reduce the cost of reviewing documents containing specific search terms, many practitioners use lower-cost contract attorneys to perform an initial review of documents that primary counsel need to explore to understand the case. The problem with that approach is that using contract attorneys to review for relevance exclusively is still too expensive, particularly when reviewers encounter irrelevant records, and duplicative when more senior attorneys must read relevant records again to verify accuracy and incorporate them into the theory of the case. In short, manual review methodologies do not compare favorably with analytics-based approaches from either a cost perspective or from a consistency or reliability perspective.
Unassisted human review poses numerous challenges that have nothing to do with the subject matter, such as reviewer fatigue, distraction, and boredom. A reviewer’s understanding also evolves and improves over time, rendering later determinations more accurate than earlier ones. Attempting to classify imprecise natural language in a document collection into discrete categories such as responsive or nonresponsive also raises serious questions about how to set and enforce a determination threshold. A number of studies have demonstrated that unassisted attorney document review can yield widely variable results, and one of the most persuasive studies was presented at the DESI IV conference. The paper describes how seven separate review teams of between six and 17 attorneys each reviewed a single set of 28,209 documents. When the results were compared, only 43 percent of the relevance calls made by the seven separate review teams were consistent. “The agreement on the responsive determination alone was 9 percent and on the non-responsive determination was 34 percent of the total document family count.”* The controlled nature of this test project suggests that the reviewers likely performed at a higher level than much larger teams of unassisted contract reviewers working for longer periods on even larger document sets. In those situations, one would expect the factors of fatigue, distraction, and boredom to have an even greater effect on the quality of the reviewers’ work.
* Faster, better, cheaper legal document review, pipe dream or reality? Using statistical sampling, quality control and predictive coding to improve accuracy and efficiency by Thomas I. Barnett and Svetlana Godjevac; www.umiacs.umd.edu/~oard/desi4/papers/barnett3.pdf
A decade ago, software and service providers began offering technology-based solutions under the umbrella term “analytics” to address the growing challenge of discovery review. These approaches came with various labels like “predictive coding,” “concept clustering,” “concept searching,” and more recently “Technology Assisted Review” (TAR). Analytics were supposed to make review more effective while reducing costs and time spent on review. Unfortunately, the concept of analytics has been long on promise but short on real cost savings in legal discovery. There are several reasons the savings haven’t been realized. “Analytics” has been marketed as a one-size-fits-all approach that adds new technology to solve discovery problems with the push of a button. Accordingly, few attorneys have taken the time to develop the deep skills needed to understand and effectively apply the wide range of ATA technologies and strategies available. When new technology is simply applied as an add-on to existing review processes, the result is confusion and unmet expectations among the attorneys and legal services firms who use the technology. New technologies usually require new processes applied by those who understand the tools’ capabilities and limitations to achieve real improvements in performance and reductions in cost. If the new technology is just “layered onto” the existing process, it becomes an additional cost rather than providing savings or even significantly meaningful results.
In the pre-digital era, limits on the number of records that lawyers would have to read for discovery review were uncommon, and largely a function of a practitioner’s negotiation and advocacy skills. In the past decade, discovery limits have become more common and necessary as ESI has gained acceptance as the predominant form of text information involved in litigation and investigations. Text search terms to select only documents that have a reasonable likelihood of being relevant have been the most widespread way to reduce the number of documents requiring review. Search terms are not generally considered “analytics,” but they can be highly effective. Because they are generated and evaluated manually, search terms can be laborious and expensive to develop and defend. Achieving consensus between parties or judicial approval on search terms is also often more art than science. Moreover, the search term development process can be severely hampered if parties fight about every issue, or if there is a large gap in technical sophistication between them and/or the tribunal.* Seemingly simple issues, such as the acceptable rate at which the search terms fail to identify responsive documents, can become fraught with complexity as statistical or linguistic challenges are raised.
* The Sedona Conference Cooperation Proclamation is an excellent exposition of the problems, and solution, for when litigants fight about every issue, rather than picking their battles. The problems created by insufficient cooperation and technical sophistication in limiting discovery are endemic and not restricted to search term development.
Analytics tools to reduce review volumes
In recent years, the analytics approach known as TAR has been used and judicially affirmed* as a viable document review reduction strategy. TAR, which belongs generally to a group of machine-learning algorithms known as “classifiers,” helps practitioners rank and classify documents according to likely relevance, privilege, or other issues. The ATA approach of TAR can be defined in several ways, but the most popular explanation is that it uses “human in the loop” reviewers to direct a method called supervised learning. These reviewers make decisions about samples of documents, selected either at random or by more complex algorithms. These decisions “train” a model to learn how to recognize document features associated with the review goal, such as identifying relevant facts. Once training is complete, the model categorizes the rest of the document population consistently with the training set, usually by assigning each document a score that indicates how strongly a document is correlated with the goal of the model. These scores can be used to order documents according to rank to either prioritize further review or establish thresholds below which only limited (or no) review will be performed.
* The shot heard ‘round the world, providing the first judicial imprimatur for predictive coding was Da Silva Moore v. Publicis Groupe, 2012 U.S. Dist. LEXIS 23350 (SDNY, Feb. 24, 2012).
Almost all TAR techniques have the potential to more fully leverage the work of a knowledgeable reviewer or team of reviewers by both identifying relevant information more quickly and ranking that data in order of relevance. This kind of ATA review tool is the most recent development in the analytics continuum and if properly deployed, offers the greatest productivity impact for typical review in terms of cost performance. TAR is not only applicable to cases within the United States but also internationally. Machine learning provides cost and time savings for tackling large data volumes in almost any context. Common law recognizes the benefits of efficiency in administering justice, so TAR has been favorably treated in international courts.
More recently, a master in the English High Court in the Pyrrho Investments matter approved the use of predictive coding in identifying documents for disclosure. He cited several reasons for his decision including among others: 1) it has been found useful by courts in other jurisdictions; 2) there is no evidence that it leads to less accurate disclosure than the use of manual review and keyword searches; 3) applying the judgment of a senior lawyer through a computer algorithm can be more consistent than having dozens or hundreds of individual reviewers try to apply the relevant criteria to individual documents.
Why international courts are ruling favorably on the use of TAR and predictive coding
Last year in the case of Irish Bank Resolution Corporation Limited & ors v Sean Quinn, the Irish High Court ordered that TAR in the form of “predictive coding” complied with the court’s rules regarding discovery. Beyond citing Judge Peck’s order in DaSilva Moore, the court noted that “…in discovery of large data sets, TAR using predictive coding is at least as accurate as, and probably more accurate than, the manual linear method in identifying relevant documents.” Additionally the court said, “If one were to assume that TAR will only be equally as effective, but no more effective, than a manual review, the fact remains that using TAR will still allow for a more expeditious and economical discovery process.”
Analytic tools to improve review productivity
While TAR can eliminate many documents from further review, reading documents is an unavoidable necessity for understanding content, and ATA approaches can dramatically influence this rate of review. Among the first productivity tools to be applied to improve legal document review speed was clustering based on similarity in text features and patterns. The first clustering tools were unsupervised, meaning that the software algorithms automatically brought like documents together into groups or clusters based on the words they included, without the need for human input. The most popular early clustering tools were “near duplicate detection” and “email threading.”
Near duplicate identification is used to find all versions of documents in a population with similar text content, such as different versions of a contract. The near duplicate detection process groups similar documents together and highlights differences between them. This approach permits reviewers to avoid a full re-read of sometimes lengthy documents by learning the content of one version and focusing on differences in near duplicates.
Email threading, which is also unsupervised, identifies all components of an email conversation based on a common first message and pulls them into “email threads” so that all emails for a given conversation are grouped together. More advanced implementations identify the longest and most inclusive emails within a chain, and suppress the shorter emails so that a reviewer can limit review to only the longest, most inclusive emails. Collectively, near duplicate detection and email threading increase review productivity and consistency because document groups are presented with greater context and more uniformity of content.
Supervised and unsupervised analytics can increase productivity by reducing the number of documents that have to be reviewed and grouping those requiring review in a way that makes them contextually related and faster to review. Review moves more quickly and accurately as a result. The more such tools the reviewer can increase throughout, sometimes referred to as “document decisions per hour,” the more impact they have on review cost productivity.
Emergent analytics tools
Cost productivity and review speed are the most well-known applications of ATA and other analytics, but there are other emergent analytics methodologies entering the marketplace to help legal counsel make better decisions by improving insight into data and time-to-knowledge. These techniques work particularly well with the growing focus on “data visualization,” which is an entire discipline of data analytics focused on ways to make data analysis more intuitive and easy to work with. Social Network Analysis (SNA) is one useful method for investigating individual behavior by showing how people communicate. By leveraging graph theory, SNA graphically displays communication frequency between people, and can often be filtered and manipulated to see who is talking with whom, about what, and when, which allows investigators to determine quickly how a person of interest might be communicating with others about a particular topic. When tied with the powerful data visualizations that have become the hallmark of modern analytics tools, problematic communications often can be identified in very little time. Moreover, using techniques like “two-hop” routing, it is much easier than ever before to identify classes of potential custodians, or to rule out others, using a straightforward and clearly explainable methodology. The power in using association-based analytics like SNA is that practitioners can see associations between events, people, and times in ways that were not as clear in a predigital world. More than classification and unsupervised learning techniques, SNA is a very useful approach for drilling into a large data set to spotlight areas of potential importance.
Yet another area where analytics builds insight is in creating new categories that were previously unavailable. “Sentiment analysis” is a popular example of this kind of ATA approach. Sentiment analysis identifies patterns of language within a document to determine the kind and degree of emotion associated with it. Sentiment scores and categories can be used as another way to slice document populations into groups for further analysis or review, particularly when analyzed against other key features, like time or author. These can be used to drill down quickly into intriguing and unexpected patterns of behavior, such as an employee’s angry communications that involve particular work events that might suggest a motive to commit a crime or other wrongdoing. For example, a search term for a company’s competitors might yield too many documents to review quickly, but combining that search with a sentiment score can allow a reviewer to start with documents where that company was discussed in an emotionally charged way, which might lead quickly to useful insight.
ATA tools come in many different forms, and can be deployed for a variety of purposes. That said, they are subject to different and often-confusing pricing models, which can be difficult to understand and compare. Software costs are usually on a per document or per data volume (usually gigabyte or GB) basis, but can also be found on a per user or per year basis. Since no technology runs itself, additional training and support is usually needed. Consulting support and training may be included in the software cost, but may also be provided on an hourly basis.
Achieving the highest quality review results at the lowest cost requires detailed knowledge of the review needs, the strengths of each of the various ATA tools, and a well-designed process that includes sampling the review results to confirm that the tools have delivered the desired level of quality. Although the legal profession is cautious when adopting new tools and approaches, as these newer tools are more fully vetted, they will be more widely adopted, particularly as the field of data science becomes more accepted in the business world. The scholarship underlying many ATA techniques is decades old, but uptake in the legal industry is still nascent. Impact on review quality and costs will become more widely appreciated in the coming years, leading to increased acceptance and use in the legal industry.
International considerations
The need for better and faster handling of ESI extends beyond litigation document review for discovery in the United States. Understanding a company’s data and getting in front of potential problems is a compelling need for international businesses as well. Data privacy, particularly in the area of personal information, is a growing concern for international entities, since breaches of privacy protections can result in fines of up to five percent of global revenue. Systems that are trained to monitor and alert potential compromises can stop very expensive data privacy problems before they escape beyond a corporate firewall.
Another area of interest to international practitioners is early identification and routing of documents in different languages. Every situation where a reviewer encounters a record in a language they cannot read is an avoidable inefficiency. Technology-based solutions can address these issues before they arise by detecting and assigning documents according to primary language (False positives and negatives in language detection are unavoidable, but can be minimized through careful attention to language detection settings). The cost of poorly routed foreign language documents can be considerable. Foreign language review expertise is typically more expensive than English language review, so every English document routed to a foreign language reviewer represents an overcharge for labor. Conversely, every foreign language document mistakenly assigned to an English language reviewer must be tagged and routed manually to a foreign language resource, which takes expensive review time to perform an administrative function. The cumulative effect of taking these corrective actions for a sizable review can translate into thousands of dollars of avoidable costs.
Technology assisted review for small law departments with small case loads
For small corporate law departments that don’t have ongoing large litigation with large e-discovery demands, Technology Assisted Review (TAR) can still be a way to help keep the small litigation budget small. An in-house lawyer responsible for responding to e-discovery requests in a couple of recent cases worked for a company that had two unrelated cases — both including requests for production of all emails and other documents relevant to the issues in the case. In each case, the universe of potentially relevant documents numbered in the tens of thousands. In the first case, a commercial claim in which the company was the plaintiff, the entire volume of potentially relevant emails was turned over to outside counsel for review and production. The initial review of all those emails was assigned to a junior associate of the firm, whose job was to identify all the relevant emails for the partner trying the case. That review was highly expensive for the company and produced only a small number of relevant documents. After that experience, the company vowed to use a more efficient approach the next time it faced a large e-discovery request.
The next time came shortly thereafter in the second case, a premises liability matter. Like the first case, discovery included another document request for all emails and documents relevant to the issues in the case, and again the universe of potentially relevant emails numbered in the tens of thousands. This time, the in-house counsel decided to do her own initial review of documents using TAR. The company retained an e-discovery firm that used an analytics tool that allowed her to train the system on the types of emails that would be relevant to the request for production. She did the training herself, because she was most knowledgeable of the case and the issues. Her knowledge of the case helped the system learn quickly and efficiently. After several rounds of training, it became clear that the system’s algorithm had identified virtually all of the relevant documents. After that, the company only had to further review those documents where some question of relevancy still remained, and for most of them the final answer was not relevant. The entire process, including all charges for the TAR, was done at a fraction of the cost of the email review in the first case, saving money and improving accuracy.
Getting started
Whether domestically or abroad, the first step in moving toward analytics-based approaches is to define goals and tasks required clearly and — to the extent possible — quantitatively with metrics to address accuracy, cost, and time. No evaluation of analytics can succeed without establishing a benchmark for comparison. With this baseline for comparison, analytics-based approaches can be evaluated and cost-efficiencies projected. The analytics projections should be informed by the experience of other users and experts, and tailored to each organization’s specific data needs. Analytics approaches also should factor in learning curve issues and the need for expert assistance.
Approaches should only be considered if results of documents identified, produced, or withheld are “as good as or better than” what can be achieved with traditional Boolean search and manual linear review in terms of accuracy, costs, and time invested. While TAR and predictive coding technologies are relatively new, the long-standing paradigm of “People, Process, and Technology” — in that order — still applies when implementing TAR or any other technology solution.
A breadth of skills in e-discovery, data science, and statistics is critical. Since analytics in the legal industry is an emerging need, most companies and law firms have not yet fully internalized all the necessary skills, particularly given the wide array of available technologies. This skills gap is changing, and some law firms have added data scientists who can guide their clients in applying analytics tools to their matters. There are also independent consultants with deep experience in e-discovery, data science, and statistics who can serve as guides or “owners’ reps” to in-house counsel to optimize the experience and help avoid first-time mistakes.
The discovery process, just like legal advice in general, is not “one size fits all.” Each implementation needs to be tailored to maximize the benefits of technology and minimize errors. Simply adding TAR or predictive coding software to a traditional review process will yield minimal benefits and will likely increase the costs and headache. The process has to be designed to fit the situation, document decisions, and optimize the resources required to achieve the required results.
Finally, the specific technology can be the least critical element in achieving a good outcome. The variety of analytic tools on the market can be intimidating to first-time users. So, when getting started it’s important to understand the project needs, then work with people who understand which algorithms or tools and associated processes can best support those needs, rather than selecting a technology and then trying to fit the discovery process to the tool. Many companies have brought e-discovery software in house only to find that supporting those tools at the scale required was far more demanding than anticipated. Since analytics technologies require IT support, the IT team should be involved in the selection process. But support requirements for e-discovery software are very different from the support needs of traditional business applications, so appropriate caution is recommended. Specialists in e-discovery can be very helpful in supporting the company’s IT team in selecting a technology that will fit the requirements and resources. There are also many outsourced discovery providers whose businesses are designed to support the vagaries of e-discovery in a variety of ways, from behind-the-firewall implementations to fully hosted solutions accessible through cloud environments.
Conclusion
Just as the world adapted to take advantage of the availability of printed material made possible by Gutenberg, the legal market will come to understand the productivity and quality differences new text analytic tools can deliver. Traditional review methods will give way to higher-value approaches that translate directly into competitive advantage within the legal landscape. As the broader business intelligence market has demonstrated, the legal industry will benefit when it revises its processes and methods to reap the advantages that advanced text analytics can provide. So, start by finding the right people with a breadth of analytic and data science experience to guide your successful application of these powerful tools.