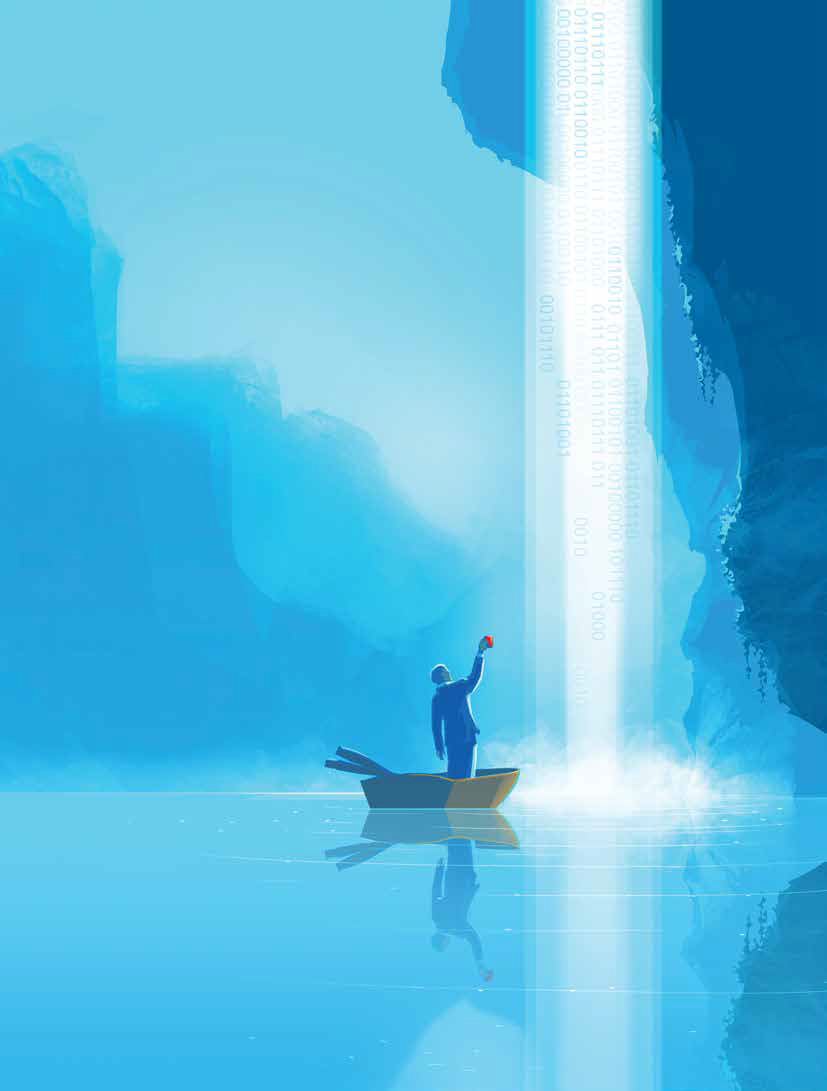
CHEAT SHEET
- Stay abreast of local intellectual property laws. The explosion of the market for big data and associated analytics has been mirrored by a proliferation of laws and regulations, based on the server location.
- A dark side to “enriched underwriting.” UK regulators are considering barring insurers from clandestinely scraping a variety of sources of data to evaluate consumers. Other regulators may follow.
- Regional differences abound. One of the many disparities between US and EU regulators is stringency of anonymisation. American regulators offer a more lenient definition of anonymisation for big data analytics.
- One big tent. The interrelated nature of IT, security and privacy policies in a regulatory context has motivated some companies to create a single data policy for compliance purposes.
“Big data will be transformative in every sphere of life.” This is not a slogan promoting a Silicon Valley start-up, but the White House’s assessment published in May 2014. With rapid advances in IT and communications technology, we are getting better at extracting insights and value from information. This, in turn, is driving the monetization of data as a commodity. As a result, we are seeing the evolution of parallel but competing pressures in technology deals and transacting norms. On the one hand, information is becoming the customer’s crown jewels — not just valuable or sensitive, but a core commercial asset. On the other, vast data storage, processing and analytic capabilities used to realize that value are becoming cheaper and more accessible to those customers in a highly competitive and flexible online computing environment.
This article looks at five trends emerging from the “big data” industry:
- The evolution of intellectual property laws to give greater protection for data and data sets;
- The increasingly complex arena of regulation around the exploitation of information, across industries and territories;
- How businesses are adopting operational measures to minimize the risks associated with big data processing and exploitation;
- How these trends are driving new transacting norms in technology deals; and
- The role analytics technology will play in commercial risk assessment.
Big data
Big data technologies can collect information at huge scale from any data source — in both structured and unstructured formats — and analyze it at high speed to deliver commercial and operational efficiencies, as well as business insights supporting deeper strategic decision-making and predictive risk assessment.
Along with the quantity and speed of information processing, some solutions aim to reduce uncertainty in the integrity of the information being captured. This is a particularly sensitive issue where the data user is handling information identifying living individuals.* A common theme in privacy rules around the world is the central requirement for personal data to be kept accurate and used only in ways that are consistent with the legitimate expectations of the individuals concerned, with information security being paramount.
* “Personal data”, “personally identifiable information” or “PII”.
International Data Corporation’s 2015 predictions conclude that the overall big data and analytics market worldwide will reach $125 billion this year, with spending on rich media analytics set to triple. The value of data in all its forms is reflected in the expanding landscape of laws and regulations protecting information.
The intellectual property in data
Big data is typically amassed by using webcrawlers (sometimes called ‘bots’) programmed to scrape information from many websites. Scraping can lead to IP claims by website owners for misappropriation of their web content.
Information is not property
Where the target information is publically available, it is unlikely to be protected either as confidential or as property per se, meaning that the legality of webscraping (which crucially entails copying) depends on whether any IP right can be attributed to the information in question.
Relevant IP rights
For data mining (an inherent component of many big data projects), there are largely three different kinds of IP rights to consider in Europe (the law derives from the Copyright Directive and the Database Directive, both applicable across the European Union ):
- Copyright in literary works: copyright may subsist in a literary work, which can be as minimal as a sentence, or all or part of a blogpost, provided that it is original (meaning that the work is not itself a copy). Copying all or a substantial part (in a qualitative sense) will constitute infringement.
- Copyright in the database structure: Copyright will subsist in a database* only if it “by reason of the selection or arrangement of their contents, constitute[s] the author’s own intellectual creation.” Effort and skill in connection with the content are irrelevant. Copying all or a substantial part of the database structure would constitute infringement. There is an exception for a lawful user to carry out otherwise infringing acts if they are necessary for the “purposes of access to the contents of the databases and normal use of the contents.” This right cannot be excluded by contract under Article 15.
- Database right in the contents: a database right subsists if there has been “substantial investment in either the obtaining, verification or presentation of the contents” of a database. Any investment made in the creation of data is irrelevant. Extraction of all or a substantial part (in a qualitative or quantitative sense) of such contents will infringe, as will repeated and systematic extraction of insubstantial parts, if it unreasonably prejudices the database right-holder’s legitimate interests. Under Article 8 of the Database Directive, a lawful user of a database may extract insubstantial parts of the contents. This right cannot be excluded by contract under Article 15.
* Defined in the EU Database Directive as “a collection of independent works, data or other materials arranged in a systematic or methodical way and individually accessible by electronic or other means”.
Both the Copyright and Database Directives provide an option for EU member states to include an exception to infringement where acts are carried out for non-commercial research, with a current proposal to make it mandatory across the European Union for the purposes of data mining.
When is EU law applicable?
EU law will apply if copying or extraction takes place in the European Union, which may mean no infringement occurs if the data miner’s server is based outside the European Union. For example, if the web content were transmitted to a server outside the European Union where the scraping actually takes place, a miner’s argument would be that there is no breach. However, it is at least possible that the EU courts, seeking to protect EU-based website content, may take a wider view of what constitutes infringement.
Contractual restrictions provided for in a website
Most websites have terms and conditions that users are expected to comply with when accessing and using content. For example, provisions on governing law or terms prohibiting copying or extraction of information by automated means for commercial purposes. Such terms are more likely to be enforceable contractually (or as a license subject to restrictions) if the user has to log in or accept them before accessing the website.
Website owners could also adopt the Robots Exclusion Protocol, by including a short command to deter unwelcome webcrawling. The lack of such a command has been held to constitute an implied webcrawling license in the United States, although the position in the European Union remains untested.
If a set of data held in a website is unprotected by copyright or database right law in Europe, is it in a worse position to prevent webscraping when compared with a website whose data are so protected? Surprisingly, a recent decision by Europe’s highest court (the Court of Justice of the European Union) found the opposite, deciding that the exceptions stipulated in the Database Directive (allowing lawful database users to copy or extract insubstantial amounts for access and normal use) do not apply to databases which are not protected by copyright or database right. This is significant because it is common for a database to be devoid of any IP rights under EU IP law. In such a case, from the website owner’s perspective, restrictions on webscraping assume even more importance.
Comparison with US law
The test for copyright infringement is similar to Europe, although there are important differences:
- A database right does not exist, although a creatively arranged compilation may qualify for copyright protection. Factual data may not so qualify.
- US copyright law includes a ‘fair use’ defense, which can apply if the use is transformational, such as crawling websites to identify a trend, but only in limited circumstances.
- US law will probably apply if the target website’s server is located in the United States.
Apart from the lack of protection for factual information, the position of web scraping under IP law in the United States is similar to that in Europe.
Regulatory protection and control of data
Regulatory controls over big data include data privacy, antitrust, antidiscrimination and potentially taxation laws. Of these, data privacy laws are likely to have the most cross-jurisdictional impact (in terms of controlling exploitation of big data that includes personal data).
Big data often involves the reuse of data collected for another purpose. Under existing EU data privacy laws, any reuse would need to be ‘not incompatible’ with the original purpose for which it was collected.
The Article 29 Working Party (consisting of the data privacy regulators across the European Union ) has set out a four stage test to determine when this requirement is met, including a requirement for safeguards to ensure fair processing and to prevent undue impact on the individual.
Reuse is more likely to be compatible with the original purpose if it is impossible to make decisions regarding an individual based on the reused data, or if the data are anonymised. However, in many cases, the only way to overcome data privacy concerns is through obtaining further consents.
In Europe, a draft regulation (the “Regulation”) to overhaul the existing EU data privacy laws is currently under consideration. The primary aim of the Regulation is to harmonise data protection legislation and enforcement across the European Union. It applies the current conceptual framework for analyzing big data, but significantly increases the sanctions for non-compliance. The new Regulation will be generally applicable across all industry sectors. However, it is likely that sector-specific regulation will also emerge to regulate the use of big data where it involves consumer (personal) data or where its use creates unmitigated risk for key stakeholders within the relevant industry.
For example, big data is an increasingly important analytics tool for the insurance industry. The volume of data that can now be collected and analysed offers opportunities for “enriched underwriting.” In this context, big data analytics may enable insurers to more accurately price risk based on data gathered on a customer during the underwriting process (which might include the way a customer fills in the application form — such as the timeframe it takes to do so). Customers may not be aware of the many sources of data that insurers and underwriters might use (and which were not consciously provided by the customer) in order to assess risk, raising the possibility that inappropriate weightings could be given to possibly irrelevant data.
This may be one reason why the Financial Conduct Authority (FCA), the UK’s financial services regulator, recently announced that it is conducting a market study into how insurance firms use big data. As part of its market study, the FCA may examine whether such an approach is contrary to Principle 6 of its Principles for Businesses, which requires that firms treat their customers fairly.
Depending on the outcome of the review, the FCA may also introduce specific consumer protection measures for the use of big data in underwriting.
The sharing of data within a particular industry for purposes of big data analytics may also attract the attention of competition authorities because, for example, it might lead to the harmonization of pricing among competitors. Depending on the jurisdiction and applicable law, intervention by competition authorities (or aggrieved competitors) might also be a risk where “anti-competitive foreclosure” arises:
- From aggregations of large data sets that enable a business to act independently of its competitors and customers (in terms of setting prices and other commercial terms); or
- In circumstances where the business possessing a data aggregation refuses to allow a competitor access to it.
There is little direct precedent on these possibilities yet.
In contrast to Europe, the United States generally has a more fragmented approach to the regulation of personal data. There is currently no generally applicable data protection law applying across all industry sectors and states. Much of the big data industry is not directly regulated.
One of the oldest forms of big data in the United States — consumer credit reporting — is heavily regulated by the federal Fair Credit Reporting Act (FCRA) and its state equivalents. The FCRA regulates the collection and sale of data when the information is collected or used for FCRA purposes, which include employment, credit eligibility and other purposes.
However, when the same data are collected and used for purposes other than those regulated by the FCRA, such big data activities are not regulated. Accordingly perhaps the largest part of the big data market in the United States — focused on marketing and understanding consumer behavior — is outside the scope of the FCRA, and is therefore unregulated. In the absence of such regulatory control, the industry has been active in establishing self-regulatory mechanisms, including various online behavioral advertising guidelines that have been developed and widely adopted in the United States.
Because the same data can be within and outside FCRA purposes (depending, for example, on the purpose for which it is sold), the line between regulated and non-regulated big data activities is often not clear. The US Federal Trade Commission (FTC) has nevertheless been vigilant in taking enforcement action against infringing companies. The FTC has also repeatedly advocated regulation of non-FCRA big data on the basis that big data enables discrimination in ordinary, everyday activities.
Another key enabler of big data analytics in the United States is a flexible definition of anonymisation in US laws. Europe, by contrast, employs more a stringent definition of anonymisation, rendering data anonymised to European standards of more limited use for big data analytics.
For example, both the US federal financial privacy law (the Gramm-Leach-Bliley Act (GLBA)) and the US federal health law (the Health Insurance Portability and Accountability Act 1996 (HIPAA)) impose restrictions on use and disclosure of financial and health information, respectively, but both Acts put anonymized data outside those restrictions:
- The GLBA does not apply to information “that does not identify a consumer, such as aggregate information or blind data that does not contain personal identifiers such as account numbers, names, or addresses”;
- The HIPAA permits data to be considered anonymised if a “qualified statistician determines, using generally accepted statistical and scientific principles and methods, that the risk is very small that the information could be used, alone or in combination with other reasonably available information to identify the subject of the information.”
This is in contrast to the position under the European standard for anonymisation, which typically considers that data are anonymized only if reidentification is effectively impossible. That difference in approach means that, unlike Europe, in the United States record-level financial data and health information can be used for big data analytics (provided the data meet the fairly flexible anonymisation standards prescribed by US law).
Piecemeal regulation in the United States (varying across sectors), together with a changing European regulatory environment, presents significant challenges for multinational businesses and their suppliers that wish to put in place long-term, multijurisdictional arrangements in relation to big data projects involving personal data.
Operational risk mitigation
Data policy
For many years, businesses have been maintaining their own IT, security and privacy policies. CIOs are now worrying about the protection and regulatory compliance of information itself as it flows into, through and out of the organization. Some companies are now revising their disparate compliance rules into a single data policy that seeks to cover all these concerns where they overlap.
Inevitably, customers and suppliers are coming into conflict over whose data policy should apply when information flows between them — especially in the context of analytics services delivered online (or “AaaS”*). Supplier data compliance teams are bringing together legal and privacy counsel alongside technical security experts, to develop internal principles-based negotiation playbooks and compromise positions so that policy conflicts with customers can be quickly resolved.
* AaaS or Analytics-as-a-Service.
HIPAA compliance
Under the HIPAA, data privacy and security rules are imposed on entities accessing the protected health information of individuals, including service providers (“business associates”) who process that information on behalf of the covered entity. A contract must be put in place between the covered entity and the business associate — a “business associate agreement” or BAA — and the regulations provide guidance as to what a BAA should include, as well as sample provisions.
It is common for covered entities and service providers to have their own approved BAA templates, so what some data policy teams are now doing is to develop principles-based internal guidance allowing alternatives to their corporate standards, provided that the substance of the final BAA is materially in line with the official version. This focus on substance rather than prescribed text gives businesses a way to fast-track policy conflicts with their counterparties.
Insurance
Data security in the context of big data analytics presents opportunities for the insurance industry. For example, the US insurance market has been quick to recognize the commercial opportunity around big data risk mitigation. The insurance industry underwrites risk by carefully assessing the frequency and severity of risk events. Through this analysis, insurers are able to consider where individual organizations are most likely to reside on a tailored “data risk map,” which charts specific risk events (like crime or network interruption) against the axis of probability and severity.
After the initial risk analysis, specialist insurers can offer practical advice on risk controls and response arrangements following a spectrum of best practices. In the UK for example, the government in 2014 jointly launched with the insurance industry a cyber essentials scheme setting out all the basic technical controls organizations ought to implement in order to mitigate cyber-risks. At the other end of the spectrum there are more comprehensive security frameworks to benchmark an organization’s level of risk — for example the National Institute of Standards and Technology (NIST) Cyber Security Framework.
There is also, of course, classic after-the-event mitigation in the form of financial cover. In the well-publicized Target data breach incident in the United States, it is reported that the company was able to recover at least $90 million of its $235 million gross expenses attributable to the breach, by virtue of specific cyber liability insurance.
Configuration of analytics tools: compliance by design
Some big data processing tools can remove privacy concerns at source through data anonymization. This can be easier said than done, since privacy rules will apply even where the data may still identify a living individual when combined with other data available to the data user.
Analytics solutions can be built with compliance in mind. User settings may be configured to ensure not only anonymization, but also that data are filtered and stored in accordance with customer policies. This is particularly key in the financial services sector. Large banks who want intelligent, searchable archiving for their regulated information* can turn to a small group of big data service providers offering solutions that the bank is empowered to configure in line with designated processing and storage rules reflecting both regulatory requirements and customer-specific policies. The technology puts more compliance control in the hands of the customer, even when the information is being processed and stored offsite via the cloud.
* E.g. SEC or FINRA-regulated in the US
Emerging transactional norms
Dealing with data policy conflicts
Alongside the principles-based negotiation playbooks discussed earlier, businesses are looking to deal with the challenge of data policy conflicts through a range of contracting solutions:
- Reliance on industry standards;
- The master policy framework; and
- Working together through change control mechanisms.
Industry standards
A common approach is to reference industry standards and codes of practice to provide a common framework to which the parties can subscribe contractually.
ISO 27018, for example, is a code of practice for protection of personal data in public clouds. It is primarily aimed at data controllers — typically the customer — but many cloud service customers are now using the standard as a requirement in RFPs for suppliers of AaaS offerings.
The challenge with international standards is that there are a number to choose from and each is subject to different interpretations — particularly between customers who will opt for the “gold-plated” approach and the vendors who need to balance literal interpretation with the need to drive down costs in order to remain competitive.
Master policy framework
An alternative and emerging approach is to use a master data policy framework. This allows the customer to be satisfied that relevant information that flows to, from and within the supplier organization does so in line with the data management rules that the customer is required to comply with. The framework can be implemented as a new contract, applying across all existing and future agreements with the supplier under which relevant data will be transacted. But applying a framework of this kind to all existing agreements in place between the parties can create revenue recognition issues for a supplier subject to certain US financial and accounting rules.
For large multinational corporations with a number of business groups, a one-size-fits-all framework contract can be extremely difficult to implement in practice. The business groups may have different operational teams who need to be involved in approving the policy terms, and even the assessment of risk will vary across the organization depending on the nature of the services provided by various divisions. The length of time and planning taken to negotiate, approve and implement the framework will need to accommodate the scale of the task.
Collaboration through change control
An unspoken reality of many deals is that neither side knows precisely what policy compliance means in practice when matching up their respective data flows and data processing architectures. So one transactional compromise — which has the dual attraction of being quick to settle and realistic about the future — is to use an “endeavors” clause, under which:
- The supplier agrees to do its best to comply with the customer’s data policy, within the overall scope of price and service stipulated in the contract;
- The customer agrees to work with the supplier to identify areas of data flow, service activity or systems operation that it does not believe comply with the policy; and
- To the extent that the supplier cannot, in good faith, achieve compliance within the service or price scope, both parties agree to use the contractual change control process to implement any changes — which may extend to service activity, infrastructure and price.
This approach involves an agreement to agree, which will prompt a chorus of disapproval from legal advisors around the world. But it is pragmatic, focused on delivering compliance in practice rather than simply ticking a box at contract signature. Compliance should be an ongoing and mutual commitment, not just a one-time hurdle.
Data analytics for legal and commercial risk management
Big data technology is enhancing risk management as a science, and will be deployed by insurance companies, law firms, in-house legal teams, commercial risk organizations and indeed any company wanting to make risk decisions based on information rather than convention.
An obvious example is the standard contract. Leaving aside official templates and precedents, all businesses have a “standard” sales contract, in the sense of the average set of terms which they sell under. But few, if any, organizations know what their average terms are. Big data solutions will provide the technological platform to deliver that information. It will be possible for a company to load its sales contracts onto a single database, to search those contracts intelligently for common positions — with meaning-based analysis rather than clumsy word-matching — and generate an authentic standard contract that is effectively the average agreement settled by the organization after negotiation with all its customers.
What this analysis will reveal is the gap between the officially approved corporate terms of business and the most common agreement that the company signs off after customer engagement. Imagine how much negotiation time could be saved by the corporation when, armed with this information, it decides to replace its official template with the average negotiated agreement approved by the corporation. Now that would be transformative.
Further Reading
“Big Data: Seizing Opportunities, Preserving Values” – Executive Office of the President, The White House, 1 May 2014.
Directive 2001/29 EC.
Directive 96/9 EC.
Article 6/1 Database Directive.
Ryanair Ltd v PR Aviation BV, Case C-30/14.
The draft General Data Protection Regulation COM (2012) 11/4.
see FCA Business Plan 2015/2016.
See for example the recent, noteworthy decision of the UK’s Court of Appeal in the “Google Safari” case - Google Inc. v Judith Vidal-Hall and others [2015] EWCA Civ 311, 27 March 2015. The Court found an arguable case that browser-generated information might constitute “personal data” when it can be combined with customer account information held by the data user (for example, because the user also happens to provide the customer with an email account).