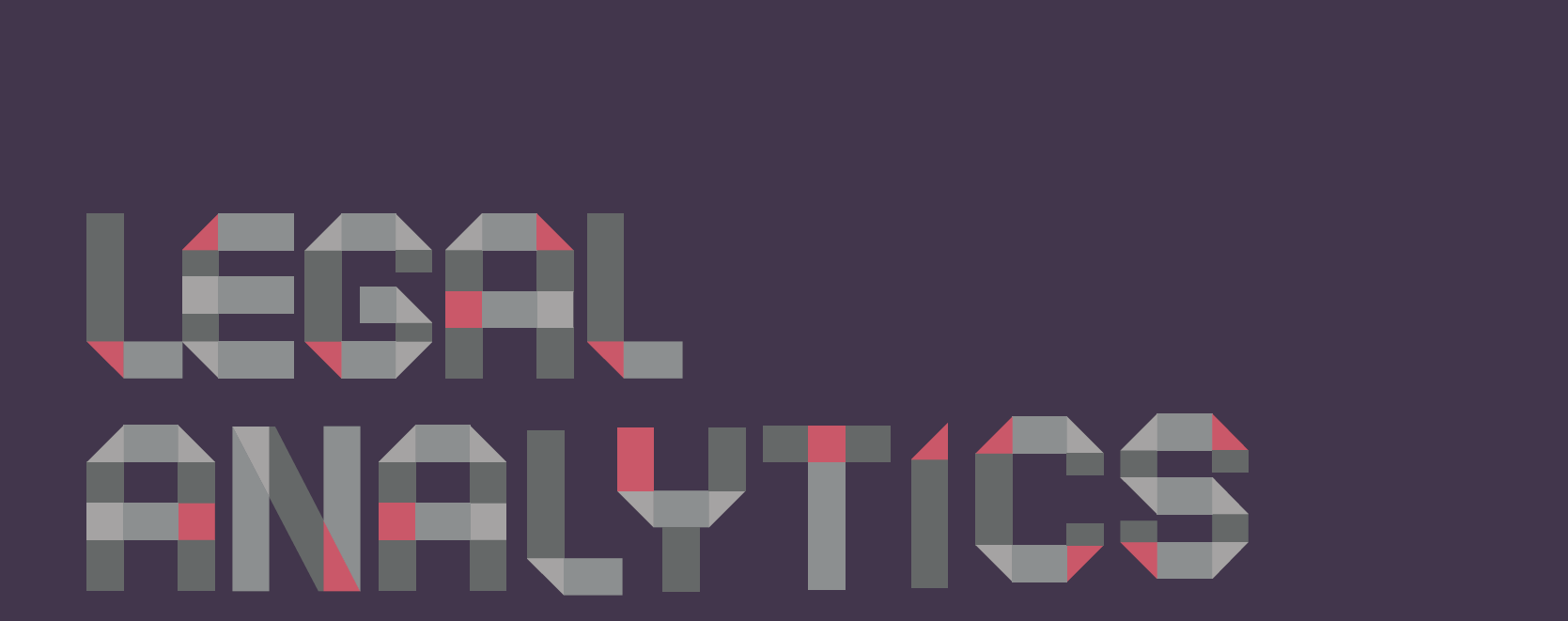
CHEAT SHEET
- Selecting outside counsel. Using analytics, in-house counsel can select attorneys that have expertise with the patents at issue.
- Managing litigation. Litigation data can reveal patterns in patent cases, which can assist with strategy and tactics.
- Evaluating judges. Determine the likely trial time and split that with the plaintiff and co-defendants.
- Evaluating the opposing party. Data about the behavior of peer companies in similar litigation can inform expectations.
Over the past 10 years, companies have moved aggressively to deploy analytics – defined as the discovery and communication of meaningful patterns in data – in order to improve management systems and produce more and better results across departments.
Human resources, finance, business development, operations, IT, engineering, manufacturing, logistics, research and development, sales, marketing and customer service all use analytics to show real gains in efficiency and performance through significant data collection and evaluation.
What about law departments? How can in-house counsel apply analytics? What data sets are available? How are they best used?
Legal analytics
Legal analytics involve the use of data to make quantitative legal predictions that inform decisions made in both the business of law (law department operations) and the practice of law (litigation and transactions). Companies can use legal analytics to select and manage outside counsel, craft successful case strategy and drive results.
Legal analytics represents a paradigm shift for lawyers.
Lawyers can now combine insights gleaned from bottom-up raw data with traditional top-down controlling authority found in statutes, rules and court opinions. We can make datadriven predictions about how opposing counsel, or a judge, or a party to litigation or a transaction, will behave. Or, perhaps, the result of a specific legal strategy or argument.
Traditional legal research and reasoning can now be supplemented with analytics to inform the advice we give, the documents we draft, the negotiations we conduct and the arguments we make.
But legal analytics also brings lawyering back to its roots. It provides us with facts on which to base opinions – the core element of good lawyering. Objective data replaces subjective “anec-data.”
In this article we will primarily focus on examples of using data in the context of patent litigation and prosecution activity.
Data helps do three things that are central to the in-house legal function: manage risk, make good strategic choices and control spending.
Types of data
In general, in-house counsel can use four types of data to assess and improve performance 1) litigation data, 2) billing data, 3) internal data and 4) other third-party data.
This article will mostly focus on some examples of litigation data, but the other categories are worth a quick discussion.
Billing data can be captured by such tools as Serengeti Tracker, which provides online invoice processing, and Sky Analytics, which analyzes invoice data along with pooled market data and can provide deep visibility into a legal department’s spend and identify areas for potential savings. This billing data can provide some general benchmarks that prove useful in case management. For example, based on the numerous non-practicing entity (NPE) cases that Yahoo has faced over the years, Yahoo now has a good pool of data from which to assess average spend per case per quarter. Yahoo can also assess the average expected life of a case – how long it will last from filing until resolution. All of this information enables the law department to provide quarterly and annual budgets with a high degree of confidence and to evaluate settlement proposals.
Internal company data helps to provide context for many cases. For example, internal Yahoo data regarding revenue and user traffic helps quantify and assess risk management. If a software feature attracts a claim of patent infringement, and it is part of a product with little revenue and a low level of traffic, Yahoo can use that data to inform decisions about what should be realistically expected, as well as help to drive settlement. Outside the context of litigation, Yahoo uses headcount data to gauge expected levels of invention disclosures. In particular, the percentage of engineers and researchers in Yahoo’s workforce, their geographic location and the business unit where they work tends to inform the number of invention disclosures that the law department receives every year.
Finally, other third-party data, such as information provided by competitors in their annual reports, or provided by the United States Patent and Trademark Office (USPTO), is often extremely useful. For example, Yahoo has used information provided by its peer companies in their annual reports to help benchmark Yahoo’s patent filings. Assessing the filing rates of other companies in view of their employee headcount or research and development spend can provide some useful benchmarks. Yahoo uses data about the relative strengths and quality of patent portfolios held by other companies to evaluate potential sales of Yahoo’s assets. In particular, Yahoo has used information provided by Innography to evaluate certain patent transactions. Data about patent examiners at the USPTO can also be used to assess prosecution strategy. For example, if an examiner’s habits are gleaned from their prior conduct in other cases, that is useful when determining whether to spend the money to respond to an Office Action or whether to abandon prosecution.
Data about the various patent portfolios on the market is also readily available through brokers and patent aggregators. Yahoo uses this data to assess the marketplace, track risks and evaluate opportunities for purchasing patent portfolios. Data about things like word count and forward citations help evaluate the strength of Yahoo’s own patents. This data can be gleaned from various sources, including the USPTO website. Yahoo has also used data about litigation results to inform decisions about what types of patents to pursue during prosecution and how to build its portfolio. That is, knowing the characteristics of patents that were successfully asserted has enabled Yahoo to focus on pursuing similar patents in Yahoo’s own patent asset development program.
Legal analytics based on litigation data
Litigation data has become more readily available in recent years, and from multiple sources. Lex Machina mines raw data from the US Public Access to Court Electronic Records (PACER) system, the International Trade Commission’s (ITC) EDIS platform and the USPTO. Lex Machina cleans, codes and tags the data using a proprietary natural language processing and machine learning engine.
For every case, Lex Machina extracts the players involved, including the lawyers, law firms, parties and judges, and identifies all asserted patents, findings and outcomes, including any damages awarded. Lex Machina also builds a detailed timeline linking all the briefs, motions, orders, opinions and other filings for every case.
All this information is then used to create structured data sets about judges, lawyers, parties and patents from the complex, unstructured legal data found in millions of pages of litigation dockets and documents.
Simply put, the impact of litigation data is tremendous. Lex Machina’s data is formatted to provide an easy case management and workflow tool for in-house counsel. Yahoo uses Lex Machina to track the patent cases on its docket, understand recent developments, store and retrieve documents, and prioritize tasks. Having that information ready has allowed Yahoo to forego weekly update and status calls with counsel and reduced the burden of tracking and storing case documents. As a result, Yahoo’s in-house attorneys can handle more work. In fact, using Lex Machina data has allowed Yahoo to streamline its team and have fewer attorneys manage more cases.
In addition to this practical impact, litigation data is useful for answering larger questions, such as selecting outside counsel, setting case strategy and tactics, and driving decision-making and results.
Select outside counsel
One of the most important decisions facing in-house counsel is the selection of outside counsel. Having objective data about counsel can inform that decision and ensure that it is not made merely out of habit, but is driven by efficiencies and effectiveness. In short, data can help make sure that corporate counsel select the best, most qualified outside counsel for the job.
Using tools such as Lex Machina, in-house counsel can easily see which law firms and attorneys have history with the patents at issue, the parties, the court and the judges. Yahoo researchs which counsel handle which types of cases, the roles they play in those cases, whether they went to trial, whether they tend to settle, and whether they have been successful and how. Perhaps more tellingly, by comparing dockets, Yahoo can have insight into typical case strategy and tendencies.
One special research project Yahoo conducted with Lex Machina was to identify a list of small firms with trial experience in the jurisdictions that Yahoo most often litigates, including the Eastern District of Texas, Delaware and Northern California. Yahoo wanted to make sure that it chose the best, most cost-efficient counsel. The project was driven by two competing goals. First, Yahoo needs to be as cost-conscious as possible when litigating. Second, and in some tension with the first goal, Yahoo wants to make sure that outside counsel have the skills, experience and judgment to take the case to trial and win. As a result of these goals, Yahoo decided to develop a list of alternatives to the roster of large law firms that Yahoo has previously used. Lex Machina assembled a list of 50 boutique firms that had never been adverse to Yahoo, had experience in litigating patent cases for other web company defendants and had successfully defended NPE cases through trial. Now, when hiring counsel for a new case, Yahoo reviews the list to make sure all solid candidates have been considered.
Interestingly, the real impact of this exercise has been to increase the instances in which Yahoo has decided to share counsel with other companies. Given the typical NPE tactic of suing multiple defendants, Yahoo has been exposed to many different law firms that represent peers and co-defendants. That same tactic provides opportunities to share counsel and split fees and costs, sometimes between multiple parties. The research on successful small firms gave Yahoo a certain comfort level with some of these firms that had been representing Yahoo’s co-defendants. In several cases, Yahoo has been able to take advantage of opportunities to share these smaller firms with other companies and reduce costs.
The results have been very successful. Legal analytics has enabled Yahoo to reduce the costs of litigation and lower expectations for settlement.
Manage litigation
Litigation data has also impacted how in-house counsel manage litigation after counsel selection, both at the strategic level and the tactical level.
At the strategic level, data helps companies understand the tendencies and patterns of behavior evident in patent cases and make decisions based on those tendencies and patterns.
A brief history of the NPE litigation faced by Yahoo may provide some insight. Yahoo was founded in March 1995. From that time until the beginning of 2007, Yahoo typically faced three or four patent cases, at most, at any given time. That changed radically beginning in 2007. Since that time, Yahoo has faced about 20 to 25 cases at any given time. These are typically all cases brought by NPEs. Very few have been brought by operating companies, let alone direct competitors that have been trying to exclude Yahoo from practicing an invention that is core to the success of their business.
During this period of heavy NPE litigation, Yahoo has faced more than 100 patent assertions as a defendant. Yahoo’s activities in those cases represent a fairly substantial body of experience, from which the law department can make judgments and draw conclusions.
For example, simply knowing Yahoo’s success rate on claim construction issues has led to a strategic decision to litigate longer. Because Yahoo has had good success on claim construction, and because that success can be case dispositive and drive more reasonable settlements, Yahoo now tends to litigate through claim construction.
Furthermore, many of Yahoo’s peer companies have also been sued extremely frequently by NPEs. Yahoo has reviewed their cases to identify their tendencies and patterns of behavior, which can and have been used as a point of comparison and benchmark for Yahoo’s own behavior. In fact, another special project Yahoo conducted with Lex Machina was to study the litigation tendencies of these competitors for this very purpose. Yahoo wanted to learn whether it was fighting too much or too little and whether it needed to change strategies in light of the number of cases being filed against it. Yahoo asked Lex Machina to gather data on a small set of peer companies, including the number of cases filed against them and the time to settlement or resolution in view of key events in those cases, such as the close of fact discovery, claim construction ruling, summary judgment rulings and trial. The data showed some interesting trends about how long and hard various companies in the industry were fighting these lawsuits and enabled Yahoo to refine its approach in light of certain benchmarks.
The most sophisticated companies not only use Legal Analytics to assess adverse parties, they also use it to assess their own litigation strategies.
The peer company benchmarking report that Lex Machina prepared enabled Yahoo to evaluate its strategic patent litigation behavior and compare it with the behavior of peers and competitors. By assessing patent litigation volumes, case outcomes and average duration, settlement propensities, trials, damages awards, propensity for NPE litigation and other subjects, Yahoo employed analytics to refine the strategy it deploys across its docket of patent litigation cases.
At the tactical level, data has been used to influence every step of the dispute resolution process, including early case assessments, choice of venue, evaluating judges and their tendencies, evaluating the opposing party and evaluating opposing counsel.
Early case assessments
Legal analytics can help answer threshold questions in patent litigation before a demand letter is ever drafted, sent or received.
Plaintiffs and their attorneys must analyze not only who has caused the harm and who has the deepest pockets, but also how prospective defendants will react to a claim. One obvious measure is the prior litigation behavior of a defendant. But what if the defendant has never before been sued, at least about the subject matter of the plaintiff’s claim? What about the behavior of similar defendants faced with similar claims? For example, a patent holder asserting a claim of infringement about a patent that has never before been litigated, against a party that has never before been sued for infringement, can extract predictive insights out of the litigation behavior of similar parties in similar patent lawsuits.
Similarly, defendants and their attorneys can look to data to inform their response to a demand letter or complaint. Has the plaintiff brought other similar lawsuits? If so, how did the plaintiff behave at each stage of litigation? Was the plaintiff successful? How have other defendants responded to the plaintiff or similar plaintiffs with similar claims or behaviors?
Choice of venue
Also consider the choice of venue by plaintiffs and efforts by defendants to transfer venue. For plaintiffs, establishing jurisdiction by a court with both a measurable track record favoring plaintiffs with similar claims, as well as one that moves faster and more often to a jury trial, can have more impact on the outcome of litigation than any subsequent tactics. For a defendant moving for transfer of venue, quantifying the arguments that have succeeded in the past with a specific judge can propel the case out of an unfriendly environment and into one where similar defendants more frequently prevail.
Evaluating judges
Once litigation is under way, every step in the process can be informed by data that improves a party’s chances of winning, while at the same time minimizing unnecessary legal spending. For example, in-house attorneys for a large pharmaceutical company had heard anecdotally that the judge presiding over their patent case often ruled on claim construction solely on the briefs, without holding a hearing. Before the company designed and executed on its claim construction strategy, it obtained data that revealed that the judge did rule on claim construction without a hearing over 80 percent of the time. The company then knew that it had to include all of its arguments in its brief, holding nothing back for a hearing that was likely never to happen.
Another example involves an assessment of trial time and preparation for trial. Facing an NPE case in the Eastern District of Texas, Yahoo knew that trial time would be relatively short, so time would have to be divided between direct examination of Yahoo’s own witnesses and cross-examination of opposing witnesses. Using the resources available on Lex Machina, Yahoo was able to find prior orders from the judge and determine the likely trial time that the judge would give for the case, enabling Yahoo to decide in advance how to split that time with the plaintiff and co-defendants.
Such granular data about judges can significantly impact litigation strategy. Data about average time to termination and time to trial, for example, can help a party budget its funds for a case and a lawyer budget her time.
Evaluating the opposing party
Data about the behavior of peer companies in similar litigation can confirm or cause adjustments to a litigant’s behavior. One large technology company known for its aggressive responses to patent lawsuits commissioned a comparative study of its patent litigation behavior and the behavior of 15 of its peers. Subjects studied included case volumes, case types, products at issue, venue, settlement volumes, case phase at settlement, settlement rates, chances of early settlement, number of cases stayed pending re-examination of the patent at issue by the USPTO, overall case outcomes, win/loss rates and damages awarded.
The results showed a wide variety of behaviors and outcomes, even in response to similar claims by similar plaintiffs. Some companies settled early. Others fought every claim to the bitter end. The data revealed an optimized combination of spending and tactics that would have been impossible to know and deploy without this approach.
The assistant general counsel in the patent strategy group of a large tech company wanted to know how often (and under what conditions) a competitor, including a recently acquired subsidiary, asserted its patents offensively. The report analyzed the competitor’s relevant litigation history and behavior as revealed in the data, as well as all asserted patents, providing Lex Machina’ customer with critical strategic intelligence on the cases pursued (and kinds of patents asserted) by the competitor.
For common motions, such as motions to transfer, stay or compel production, or to provide an injunction or protective order, or even for summary judgment, it can be beneficial to assemble and use statistics on win/loss rates for parties and counsel, as well as statistics on win/loss rates and time to disposition for judges.
Evaluating opposing counsel
Finally, zealous representation in litigation can now be informed by statistical insights into the caseloads and behaviors of opposing counsel. If you knew about all the cases that your opposing counsel is currently handling, and you mapped out all the upcoming filing deadlines in those cases, would you make sure to serve your discovery requests right when opposing counsel is busiest with other deadlines?
Aspirations for legal analytics
Although the existence and gathering of data has become more ubiquitous and more data analysis companies are popping up in the marketplace, the legal field is barely touching the tip of the information iceberg. There is both a vast amount of information that is left unanalyzed and a vast amount of information that remains confidential and, as a result, unable to be used.
For example, there is more work to be done in terms of analyzing electronic billing records, attorney time, case events, and correlating all of that information. Those records exist, but no service has yet married billing data and litigation data efficiently and effectively to enable in-house counsel to determine which firms and which lawyers are performing the best in which types of cases.
In addition, for the most part, settlement data and licensing data remains confidential, locked behind closed doors and unavailable to the general public. The result is that each company, litigant and party makes decisions based on a small set of data using their own experiences. Settlement data is some of the most impactful information driving litigation, particularly patent litigation brought by NPEs. Because NPE litigation is a business model, profits being made using that business model will be determinative of activity levels. Better said, the more profitable the business model, the more likely more people will engage in that activity and the more NPE cases will be filed. Shedding light on costs of litigation and the amount of money being paid to resolve these lawsuits would likely go a long way toward balancing the interests of NPEs and the operating companies they target.