Cheat Sheet
Urgency. There is an urgent need to enhance IP protection beyond contractual rights.
Intervention. Early, precise intervention is crucial to build controls and safeguards.
Ownership. Robust contracting and reinforcing the human element as an operational step will help to secure ownership through IP rights.
Balanced approach. IP rights are broader than contracting rights and their protection is critical.
This article first appeared in IAM and is reprinted with permission from IAM and the author.
As the next generation of data-driven analytics gets underway and the need to deliver value from AI system outputs becomes more urgent, smaller players are eyeing their chance to stake a claim in this multi-billion dollar landscape, which so far has been dominated by Big Tech. More than contractual rights, it’s IP rights that could give them the winning edge.
Advances in computing and the explosion of accessible, diverse, de-personalized, and valuable data sets are driving the latest AI developments. In turn, existing business models are being disrupted as companies scale up with vast amounts of structured and mature data, as well as unstructured data. One crucial issue is how to share data sets where parties are co-developing common AI machine-learning-related products and services.
Through an AI trained model (i.e., where an AI algorithm needs data to learn), smaller players can control and own their IP rights, as well as assert new business models and seek a financial return. The alternative is to remain subservient to the demands of Big Tech and their algorithmic rent for the entire scope of data sets.
IP rights may exist in the data or software under patents, trade secrets, copyright, and database rights. Each of these requires separate assessment, along with the end use of the AI trained model, particularly regarding outputs and their use. An infringement risk analysis can further identify new product features, design workarounds, and, more importantly, competitor differentiation.
IP protection should be considered before any disclosure requirements for transparency, explainability, and interpretability. This is because once trade secrets are publicly available, protection is lost. For novel features and functions worthy of patent protection, there may be options to prevent publication in the public domain during the filing process.
However, new business models must also take account of growing demands for free access to valuable data and use of open-source data toolkits. For example, several government initiatives (e.g., the UK AI Council Roadmap and UK National AI Strategy) are pushing for so-called FAIR (findability, accessibility, interoperability, and reuse of digital assets) data sharing in open licenses and standard templates for data sharing, such as for the National Health Service.
Understanding the legal implications of the AI trained model requires a collaborative multi-talented approach to ensure that robust operational measures are incorporated into the compliance process. This must involve all relevant stakeholders including development, deployment, and operations teams working together with IP lawyers (see Figure 1).
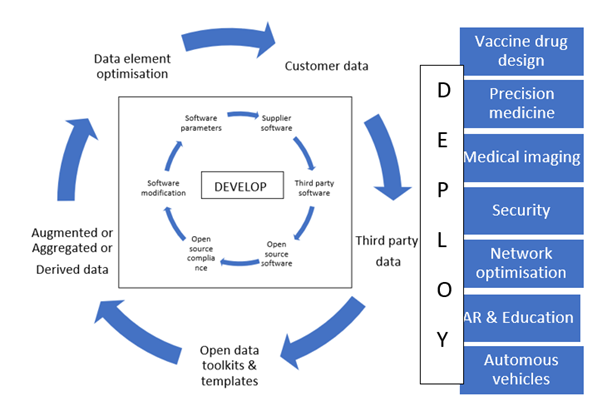
To move from structured learning based on labeled data sets to a system based on unstructured unlabeled learning (e.g., for automated decisions), businesses must open the black box – how the AI trained model works, what it learns, and how it makes decisions – and explain how outputs are generated.
This requires robust levels of scrutiny to be built into the model, such as introducing smarter labeling for data sets, thereby increasing trust in decision making. A legal team must be involved for decision making. They should introduce best practices at an operational level over the project’s lifecycle, making it easier to monitor and manage legal compliance.
Who owns what in an AI trained model
In a typical AI trained model (see Figure 2), data sets are owned by Company A and an algorithm is owned by Company B. Company B charges Company A for the use of the AI algorithm. Company A charges Company B for use of its data sets. These are used to produce a model trained algorithm for Company A. Outputs or insights can be sold to for Company A (B2B) or an end user (B2B2B or B2B2C). The AI trained model here refers to the combination of the training data, AI algorithm, model trained algorithm and outputs.
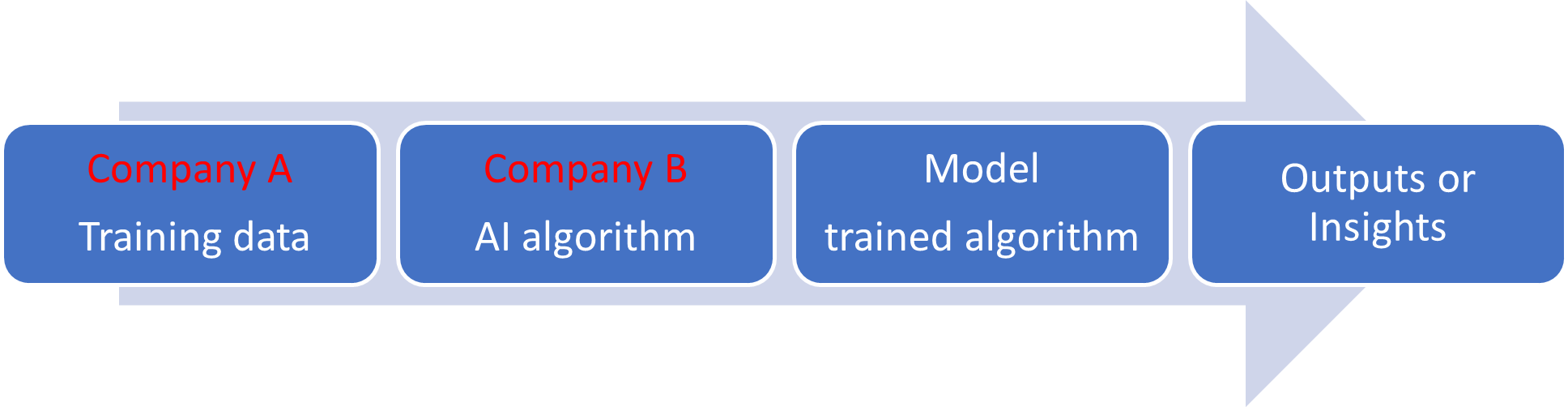
If it’s possible to place a monetary value on the data, and that the data is completely separated from individual identifiers and untraceable, the following IP issues could arise.
The training data itself needs protecting
Any data-related IP rights must be considered at an early stage in order to build an optimal data pipeline and to determine the full scope of the data sets, along with data readiness and data prioritization:
- What the core data sets comprise;
- Where those data sets originate;
- Who has rights in those data sets; and
- How the data sets are used.
The pipeline may include data from numerous sources, including third-party data, open data, text and mining data from research institutes, licensed data, and/or company-owned data.
It is therefore crucial to determine from the outset (see Figure 3) along the pipeline:
- The type of data set (e.g., real time, near real time, meta, supervised, or unsupervised);
- The category of the data (e.g., raw, aggregated, or derived); and
- Third-party (including open) data sets.
Typically, raw data is ingested, pre-processed, tested and re-tested, curated, and stored in a cloud data lake and/or in open-source machine learning software (e.g., API libraries). It is then combined with data from different sources through either aggregation or augmentation.
Derived data or second-generation data (typically owned by the creator) cannot be reverted to raw data or used to replace it. As such, data input here cannot normally be unlearned.
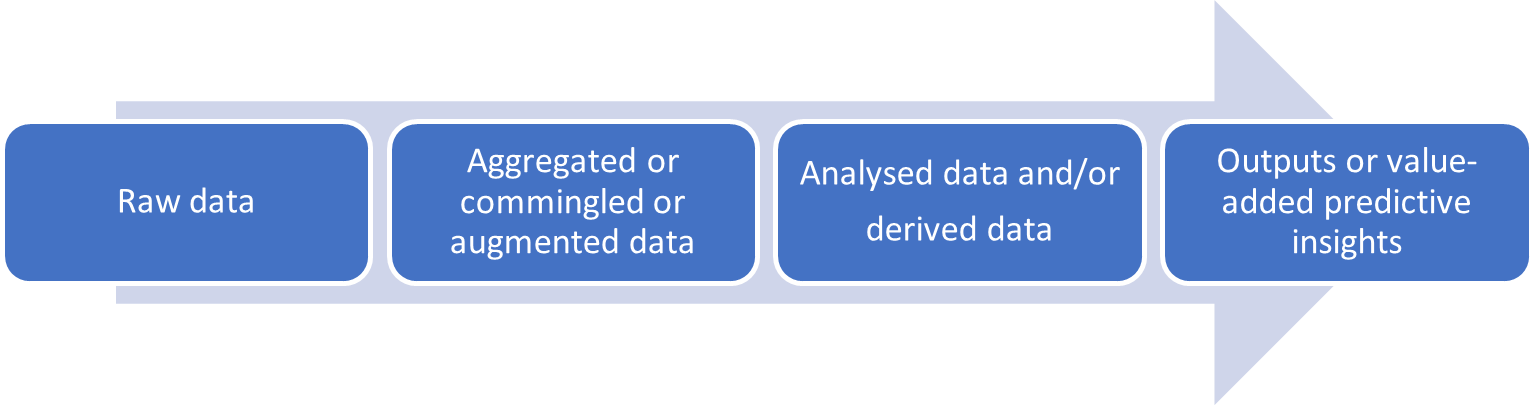
There are no property rights concerning data ownership (excluding EU/UK sui generis database rights). Further, data protection laws (e.g., the EU General Data Protection Regulation) do not mention “data ownership.” Instead, they merely refer to the data controller as the party that determines the purposes and means for processing personal data and makes decisions about processing activities.
Rights holders can carve out rights related to data with robustly drafted contracts but these are personal rights binding only the contracted parties. Thus, it is crucial to consider IP rights (enforceable against the entire world with full remedial rights) alongside contractual rights.
Training data can itself be valuable and worth protecting. There is a dichotomy between IP and data protection laws – that is, maintaining secrecy under trade secrets versus disclosure under audit rights.
While most raw data will likely lack novelty (data sets are not patentable per se), new elements or creative combinations may be considered novel and worthy of patent protection. Patents can be used to protect data sets or backroom data sets where data is part of an envisaged product (e.g., patents to software and business processes that manipulate and process data, rather than the actual data set itself). For example, tens of thousands of patents have been filed worldwide covering data processing and encoding methods.
If patent protection is not possible, or where it is likely that the data sets can be reverse engineered, valuable training data should be protected as confidential information, trade secret, or know-how. Here, company-wide processes and policies are key to show that reasonable steps have been taken to maintain the secrecy of valuable data.
Creating a secure and trusted environment where valuable data can be processed or analyzed is crucial. This could be used to establish that the information (including third-party data) has the necessary quality of confidence and that anyone with access to the data owes its owner a duty of confidence.
However, trade secrets in data cannot be shared or fully exploited without fear of copying. In this case, data sets should be prioritized, from shareable to protected trade secrets. Nevertheless, this still carries a risk that data sets may be revealed through reverse engineering.
Under UK copyright law, data set structure (e.g., whether a table or compilation) is narrowly protectable under copyright as “works” by a human. This prevents others from copying the database’s structure. However, broader rights may be obtainable under UK database rights for an intellectual creation (by a human) where data sets are assembled in the form of a database with skill and judgement used for the selection and arrangement.
This database right would sufficiently prevent others from extracting and utilizing the contents of the database. Second-generation data sets may add value to the original raw data sets and may be copyrightable in themselves.
In the United States, data sets under “works” may be protectable as compilations and derivative works (e.g., if the author chooses which facts to include, in what order to place them, and how to arrange collected data). However, the simplest compilations will lack the minimal degree of creativity required of US copyright. “Fair use” for training data sets under copyright is debatable as well as whether advances in AI warrant a sui generis IP system for data rights.
EU law (and the United Kingdom’s new sui generis database rights, created in January 2021) protects databases by copyright if they are original. Non-original databases may also be protected to the extent that third parties may be prevented from extracting and utilizing the contents where they are structured in a systematic or methodical way and there has been a substantial investment in obtaining, verifying, or presenting them. However, this right is narrow and proving investment in verification is challenging.
6 Crucial Questions About Your Data
Before choosing a course of action, rights holders should ensure that they have clear answers to the following questions:
- Are the raw data sets, derived data sets or any other components of the AI trained model best protectable as patents or trade secrets?
- If copyrights and patents are only granted to human authors or inventors, who owns or controls the use the IP in the data or software (i.e., the data producer, data curator, data user, person producing the output, original software rights holder or the person/machine making modifications)?
- From the perspective of an end user seeking to commercialise data, what is the scope of permitted licensing (i.e., who can exploit what and when) over the lifecycle of the AI trained model, given the proliferation of complicated, unclear, inconsistent contracts involved in a data pipeline?
- When balancing the interests of copyright owners in the data pipeline, what constitutes “fair use”? Is the end use or output related to a user interface (e.g., Google’s use of Oracle’s Java code)? Is this use sufficiently transformative and “fair” under Section 107 of 17 US Code? And, what constitutes derivative works for outputs?
- What is the IP infringement risk? How can it be detected? Where is the infringement evidence? If the output is publicly available, can it be reverse engineered (or disassembled)? If the output is stored in back-end servers in the cloud, how would you identify the infringer?
- Under the text and mining exception set out in Section 29a of the UK Copyright, Designs and Patents Act 1988, it is possible to make a copy of a work for the sole purpose of research for non-commercial use, but what is considered commercial use when a company wants to exploit the research-based computational analysis?
The best IP balanced approaches for AI algorithms
The AI algorithm normally undergoes swift updates in rapid agile development cycles. As such, it is not a good candidate for patent protection, given that patents are notoriously slow to grant and an application will be published 18 months after filing. Arguably, patent and data protection laws usually require some aspects of the software being disclosed to prove sufficiency or transparency.
SMEs typically invest heavily in their algorithms and keep them secret under the black box principles. They assert that competitors should not be able to understand how the machine arrives at its decisions during the processing of inputs to generate output (the black box problem). Here, the algorithm is best protected under trade secret law.
Regarding patents, lack of disclosure here may mean that the software is viewed as a non-patentable abstract idea. Further, the trained algorithm may not be sufficiently creative or inventive – both are considered human traits requiring a human actor in the chain of control. Moreover, proving infringement of software patents and the operation of AI can be challenging. All this can leave some patents seemingly unenforceable against infringers.
However, AI algorithms may be patented where functionality can be protected for novel and inventive ideas. The challenge is to select an appropriate invention for patenting and to draft suitable patent claims, which cannot be reverse engineered.
Typically, it is unlikely that training data for machine learning algorithms will need to be disclosed to show the methodology (removing the black box problem). Nor is it always necessary to disclose how the output was discovered. Moreover, a human actor is involved since all new inventions build on previous work, so the foundation of human intelligence is arguably always present.
In Europe, software can be patented as a computer-implemented invention. This covers claims involving computers, computer networks, or other programmable apparatus, where at least one feature is realized by means of a program and general methodologies. This does not involve computer programmes being claimed as concrete software code, but rather a higher level of abstraction where there is a further technical effect that goes beyond the effects generated by any computer program and that is novel, non-obvious and has utility.
In the United States, claims to AI are patent-eligible under US Code Section 101. Claims directed to an abstract idea may still be patent-eligible if the additional claim elements, considered individually or as an ordered combination, amount to significantly more than the abstract idea.
UK copyright protection is available under Section 178 of the UK Copyright, Design and Patent Act for computer-generated works with no human author. Further, Section 9(3) states that the author of a computer-generated work is the person “by whom the arrangements necessary for the creation of the works are undertaken.” The key here is to build this into operational steps into the AI trained model.
In the United States, a computer program can be protected under Section 102(a) of the US Copyright Act if it is an original work of authorship fixed in a tangible medium of expression. The work must not be copied from a pre-existing source and only needs some minimal degree of creativity. Adaptions may also be authorized.
Training complicates ownership of the model trained algorithm
IP ownership of the model trained algorithm is complicated. There are at least two moving parts as a result of the training process as the model learns:
- Training data – the elements related data points; and
- The parameters of the algorithm.
Typically, a training data set will train the complex underlying algorithms multiple times with the same algorithm while parameters of the algorithm (e.g., the number of layers in a neural network or fitness function in an evolutionary algorithm) are adjusted to optimize performance.
During deep learning development, the underlying algorithm is not usually subject to change though the parameters provided to the algorithm will change. Here the parameters represent what is learned through training, not the algorithm. The algorithm can be used with new parameters to learn afresh with new data.
Robust patent claim drafting can capture small modifications of concrete software code (and whether there are any as a result of parameter optimization) under broadly scoped claims for those modifications in the model trained algorithm. Otherwise trade secret protection should be maintained for any unique attributes for elements or parameters as the model learns.
If training does not alter the AI algorithm, copyright ownership will usually be retained by the owner or creator of the source code. However, copyright ownership in respective parties related to any software modifications based on the model learning should be considered, especially if bespoke to the model.
Patenting AI trained models
Typically, complex algorithms that underpin such AI inventions will lead to patentable technological advancements, beyond abstract ideas. Here, patents have been granted for machine-learning models or training methodologies, as well as AI-aided discoveries (e.g., for security operations, workforce management, network strength, call protection, marketing, and controlling social media).
Output predictive insights also require protection
IP ownership in output and insights – along with any other trends, recommendations, patterns, connections, or predictions – is another hot area. These insights may come in the form of a decision tree, classification, cluster grouping, a list of prioritized outcomes, or a list of factors. The full scope of permitted use cases for all outputs across different industry sectors should be considered.
Copyright ownership can be claimed in outputs where there is a human – a developer, machine, user, or employer. Where there is no human element or it is difficult to identify the creator of the works, contractual provisions should identify who performed the work. For example, a selfie taken by a monkey has been deemed not to be copyrightable.
Under Section 106 of US Copyright Law, the owner of a copyright can prepare adaptions or derivative works based upon copyrighted works. Here, it is key to ask if the output is a derivative work of the input, that is, does the work recast, transform, or adapt a pre-existing work?
In the United States, anyone who violates the exclusive right related to derivative works is infringing the copyright. In the United Kingdom, obtaining a copy of any copyrightable output – or making an adaption – requires authorization from the copyright holder. Otherwise, this constitutes infringement.
Operational AI governance
Various operational teams must take on fundamental roles across the data pipeline over the project’s lifetime to reap the benefits of AI investment. This is already happening for robust AI engineering, which facilitates the performance, scalability, interpretability, and reliability of AI models.
To understand the operational steps, as well as the role of intellectual property, rights holders must first understand how the black box works and how training occurs.
Neural networks (or deep-learning algorithms) are typically used for image processing or natural language processing. They can be trained in at least two ways: supervised or unsupervised.
Supervised learning involves teaching machines by providing them with labeled data sets and is predominantly based on structured data sets (e.g., learning a function that maps an input to an output based on examples of correctly labeled input-output pairs, before models can be used for inference).
Unsupervised learning involves teaching machines to learn for themselves so that they can make their own decisions. Machines can then act autonomously or augment human decision making, where the target label or output is unavailable, and the learning involves, for example, clustering or segmentation.
While IP rights can be used to protect the supervised learning model provided that some transparency is built in, it is less straightforward in the case of unsupervised learning, especially for automated decision making. Contractual provisions are key when labeling is unavailable and where the human element necessary for IP protection is absent.
However, new interpretable or explainability models are being used in unstructured learning to ensure that the logic of the model can be inspected, audited, and trusted, and further to explain algorithmic outputs. In this way, it is possible to train the neural networks (see Figure 4) by introducing intermediary steps or auxiliary outputs into the neural networks as cross-checks and key stepping stones or operational workflows along the way.
The key here is to determine what the neurons learn in the model and the decision-making process (i.e., how the algorithm makes decisions) before then translating this language (so-called neuralese) into English so that the output can be read by a human, thus building in a human element.
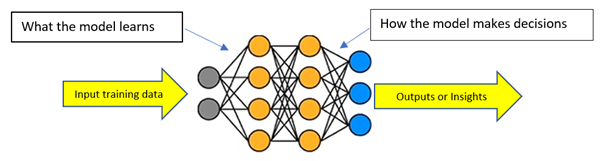
Determining human involvement
The role of IP law will depend on the operational steps implemented in the development of human-centric AI while tracking ownership in the data pipeline, such as the following:
- Identify and record all human players involved in the AI trained model, including who is controlling the machine, the role they play (e.g., data provider/curator/trainer, programmer, configurator, operator, supervisor, user, or facilitator).
- Map, label, record, and classify data flows in the data pipeline over the lifetime of the project (i.e., who owns the data, what is the core data, where it is sourced).
- Track data ownership (including third-party data) alongside purpose filters used in the GDPR, and record contributions to creativity or inventiveness.
- Identify who the last human was to make the arrangements for the creation of the works (e.g., in review or validation of the output). If no human can be identified, was the software developer the only person involved?
- Integrate human reviewers – train them to review machine decisions, to determine what logic was involved in automated decisions, and give them authority.
- Monitor the AI-trained model while it is operating – recording training methodologies, processes, and techniques used to build, test, and validate the model (e.g., by use of optimal decision trees for interpretability).
- Allow real time interventions (including right to object) by tracking and revealing AI decisions using audit trails for explainability with clear rules on when and how the machine would augment or override human decision making.
Case Studies of Ownership and Licensing in AI Training Models
A Case Study of the Licensing Model
In the first case study, Company A owns the data sets and Company B owns the algorithm. Company A and Company B cross-license rights.
Typically, Company B will have invested heavily in the algorithm (and its modifications) and assert full ownership. It will base its business model on licensing this to multiple users.
In exchange, Company A may assert full ownership in the outputs.
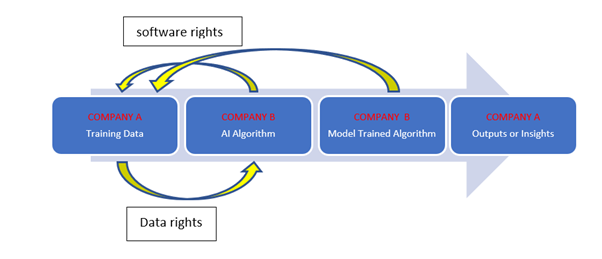
In Figure 5, Company A is granted a perpetual, royalty-free right to use the AI algorithm and any modifications. All third-party rights (including open source) must also be considered. Meanwhile, Company B is granted perpetual rights to use the data sets in relation to the model trained algorithm. The scope of the license is key particularly regarding permitted use (over guaranteed data sets or the entire scope of data sets), duration, sub-licensing, any grant-backs, and rights to outputs.
Further, how the parties are remunerated could depend upon:
- Any discounts awarded;
- Free use or preferential rates;
- The percentage of the downstream profits; and
- A percentage of the financial award.
A Case Study of the Ownership Model
In the second case study, Company A owns the AI trained model except for any underlying IP rights. This may avoid complex joint ownership issues given that property laws usually set high bars. In the United Kingdom, these include that:
- The interests of all co-owners must:
- Arise at the same time (unity of time);
- Be identical in nature, duration, and extent (unity of interest);
- Arise from the same document (unity of title); and
- Each co-owner must have an equal right to occupy or possess the entire property (unity of possession).
In the United States, Section 10 defines joint work as work prepared by two or more authors with the intention that their contributions be merged into inseparable or interdependent parts of a unitary whole. Where Company A pays in full a one-time fee for bespoke, exclusively created software provided by Company B, it may be entitled to full ownership of the model trained algorithm with free, perpetual rights to any underlying IP rights. In this case, Company A may look for contractual provisions excluding competitors from use.
Permitted scope of data and software licensing
Generating revenue from data is underpinned by the scope of permitted data and software licensing in the AI trained model, such as:
- The core data;
- Permitted purposes;
- The field of use;
- Geographical limitations;
- Internal use (or sub-licensing);
- Exclusivity (or non-exclusivity);
- Irrevocability (or revocabililty);
- Grant-back rights;
- Post-term (perpetual);
- The post-term survival of confidentiality; and
- Commercial (or non-commercial) use.
However, the data may also be governed by agreements for its open use. Account must thus be taken of any open tool data kits (e.g., the Linux Foundation’s Community Data Licence Agreement), which could be used for training data sets under a known licence model.
The future
A balanced IP approach is required where IP laws supplement contractual rights given that data protection laws do not address data ownership. Robust contracting for securing rights related to data are key. IP rights are important because they are enforceable against the entire world (not just the contracting parties) and do not limit remedies to contractual breaches only.
The AI trained model and its components are best protected under database rights, copyright, patents, and trade secrets. IP protection must be considered before any disclosure requirements. Data and its optimized elements may be valuable as confidential information. AI algorithms and their parameter optimizations can be protected under trade secret and copyright laws, while patent protection for select inventions are carefully considered.
In the absence of patenting, it is critical to show what reasonable steps have been implemented in-house to protect valuable confidential information and trade secrets, and to carve out contractual confidentiality provisions which survive contract termination.
Best practices implementing human-centric operational steps and safeguards will enhance IP rights when it is difficult to determine the scope of permitted licencing. Introducing operational steps for interpretability and explainability will help to open the black box to enable human intervention and place humans in the loop.
As machines get better at producing their own works in outputs (using automated decision making), this blurs the lines between human or machine-created works. Here the challenge is to unpick unstructured data sufficiently for human intervention. Optimized data elements combined with optimized algorithmic parameters will yield the best outputs in the AI trained model.
Early consideration is required to mitigate risk for third-party IP infringement when designing, developing, procuring, deploying, and assessing the likelihood that any components of the AI trained model infringe third-party components. This would also involve determining brand risks and suggesting design workarounds for competitive advantage.
In the United States, the interpretation of “fair use” in copyright infringement is still being awaited in Google v Oracle, a landmark case that is currently before the Supreme Court. In the meantime, early assessment of ownership of AI outputs is required since what constitutes derivative works in the data pipeline is complex under US copyright laws.
While it is debatable whether a new monopoly right in the form of new data legislation in the United Kingdom (akin to the proposed EU Data Act and EU Data Governance Act) is necessary, there are growing calls for a worldwide harmonized approach to data ownership protection, copyright, confidentiality, and database rights. With regulators developing auditing capabilities for AI systems, there is an urgent need to build controls and safeguards.
The UK government is already considering appropriate standards to frame the future governance of data. Such approaches should be viewed in line with standard templating for the non-public sector. It is also hoped that careful choices will lead to further harmonization and open sharing templates found in open-source data toolkits.
This new space offers rich pickings, particularly for smaller, more agile players. The prizes will go to those best able to maneuver through the shifting web of IP, data protection, new data laws, new data governance laws, and new regulation, to claim a piece of the new AI bounty. Intellectual property is a fundamental part of this and must be taken into consideration in all aspects of the decision-making pipeline, from design to implementation, procurement, and deployment of the AI trained model at an early stage.