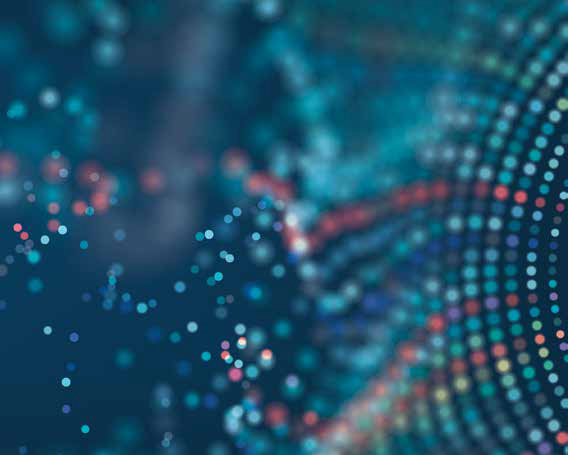
In my December 2018 column, I wrote about how litigation risk analysis techniques can be used to help evaluate litigation decisions. In this column, I will describe how data analytics may become an even more valuable and effective litigation management and prevention tool.
It has been obvious for many years that litigation data can provide valuable insights. In fact, an early metadata-classification/tagging system called the West Key Number System was developed in 1906 by John B. West. It was used to help lawyers identify relevant points of law to research as precedents, and also as guidance on how particular cases might turn out. With the advent of digitalization, Westlaw, Lexis, Bloomberg Law, and other companies have begun offering online research tools that include various kinds of data classification and analytical tools that lawyers can use to evaluate particular cases.
At first, these databases relied heavily on human editors to identify themes, points of law, or specific issues. More recently, however, we have begun seeing how machine learning and other kinds of AI can be used to harvest information about cases automatically. And, as more information has become digitally available, we have begun to develop helpful pattern recognition techniques for predicting how a particular piece of litigation will play out, as well as the best resolution strategies to apply.
This is clearly a work in progress. Early uses of data analytics in this field focused on how courts in a given state would address particular legal arguments. As more cases and information populated our databases, we were able to narrow the data down to specific counties, then to particular judges. Soon, we were able to see how particular judges might react procedurally — which judges might rule more favorably on a motion for summary judgment, and which ones might react better to the opposite: the carefully built legal argument presented in the context of selected facts.
We also became better able to evaluate expert witnesses and opposing counsel based upon their strengths or weaknesses in other cases. We could evaluate both our own case, and opposing counsel’s, and discern whether they tended to use certain strategies or tactics to pursue their ends. We could locate outside counsel in jurisdictions new to us who had specific expertise in the areas we needed. We could even identify more effective process servers in a given jurisdiction. Furthermore, we could develop a more accurate prediction of expenses associated with different types of cases. All of these examples are likely very familiar to anyone who has managed litigation for the past several decades. But we are now on the cusp of an entirely new era of litigation data analytics.
The Gartner Group is one of the world’s leaders in technology data research and analytics. The Group created a graph that illustrates the potential evolution of data analytics.
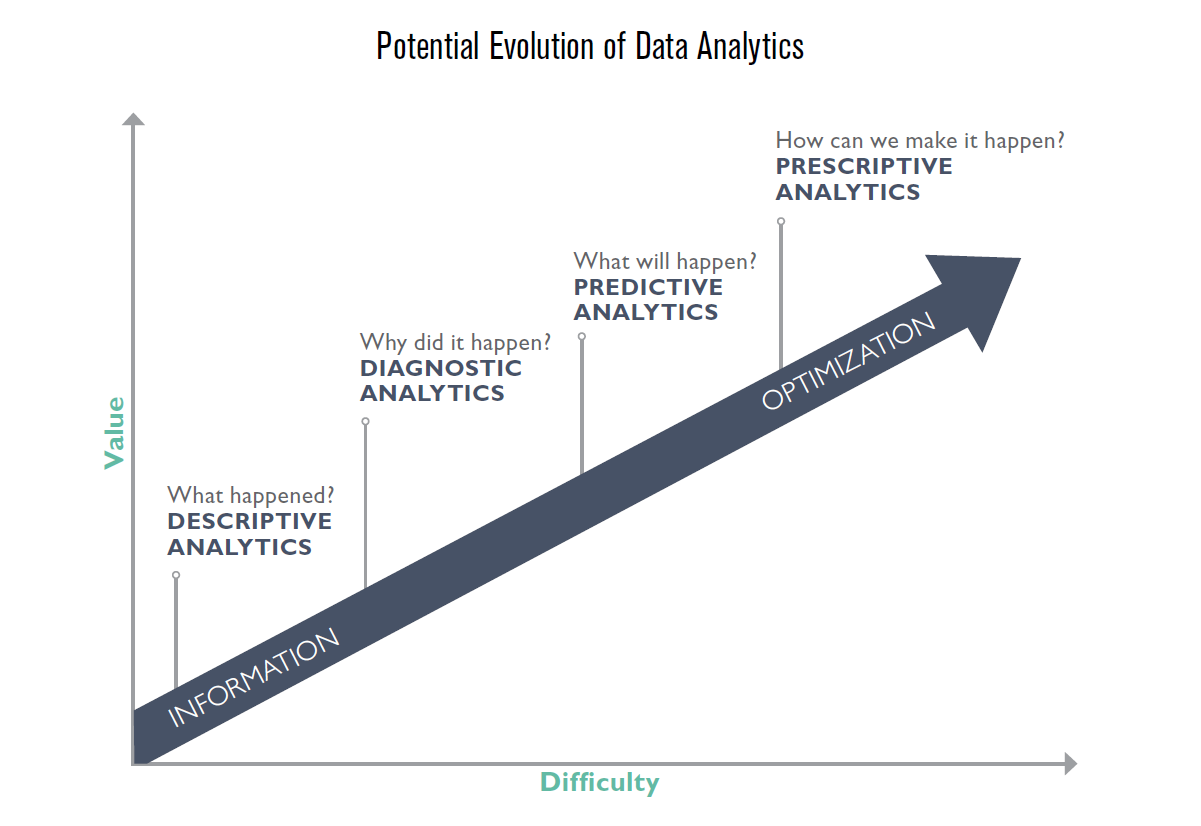
As you can see, the early stages of descriptive and diagnostic analytics help us understand what happened and why. For example, first, we can detect what patterns may have occurred in judicial interpretation (descriptive analytics), which will then enable us to better understand why the judiciary reached the conclusions it did (diagnostic analytics).
From there we can build that information to try to predict what will occur in a given circumstance (predictive analytics). “One judge loves these types of legal arguments; this other one does not. Therefore, we are likely to get this result if the case is assigned to the one instead of the other.” Finally, this information may allow us to try to influence the outcome (prescriptive analytics) by, for example, filing in one court instead of another. As indicated by the graph, the greater the value of the analytics, the more difficult it is to obtain. But as computers become more powerful and data becomes more available, it becomes less difficult to perform the analysis.
Clearly, the more data we have and the more analytics we can apply, the more effective we can become in this arena. So, for example, a recent study showed that when a motion was referred by the presiding district court judge to a magistrate judge for a preliminary report, the magistrate judge’s recommendation was the strongest predictor of the judge’s ultimate decision.
Having discovered that, we now know that we should try to track and include the profiles of magistrates in our analyses.
Data analytics can also create graphic representations of litigation that can help us make better decisions. For example, the above visualization was created by analyzing the outcomes of certain employment cases in Georgia.
Tools like this cannot only be used to help clients understand the risks they may be facing in a particular case, but also can inform the creation of litigation risk analysis decision trees for that case.
But these kinds of things are just the beginning. AI is now being used to predict case outcomes, and in at least two studies, AI has been substantially more successful than experienced litigators.
The real promise underlying data analytics is to identify correlations that humans cannot readily discern (Pun intended). I have written before about the Target situation involving a teenage girl in which the store’s analytics successfully identified her pregnancy before her parents were informed. In that instance, Target managed to correlate the purchase of a wide variety of products not obviously associated with pre-natal activity with a customer’s pregnancy. It is interesting to consider how such analytics might apply to jury selection, for instance.
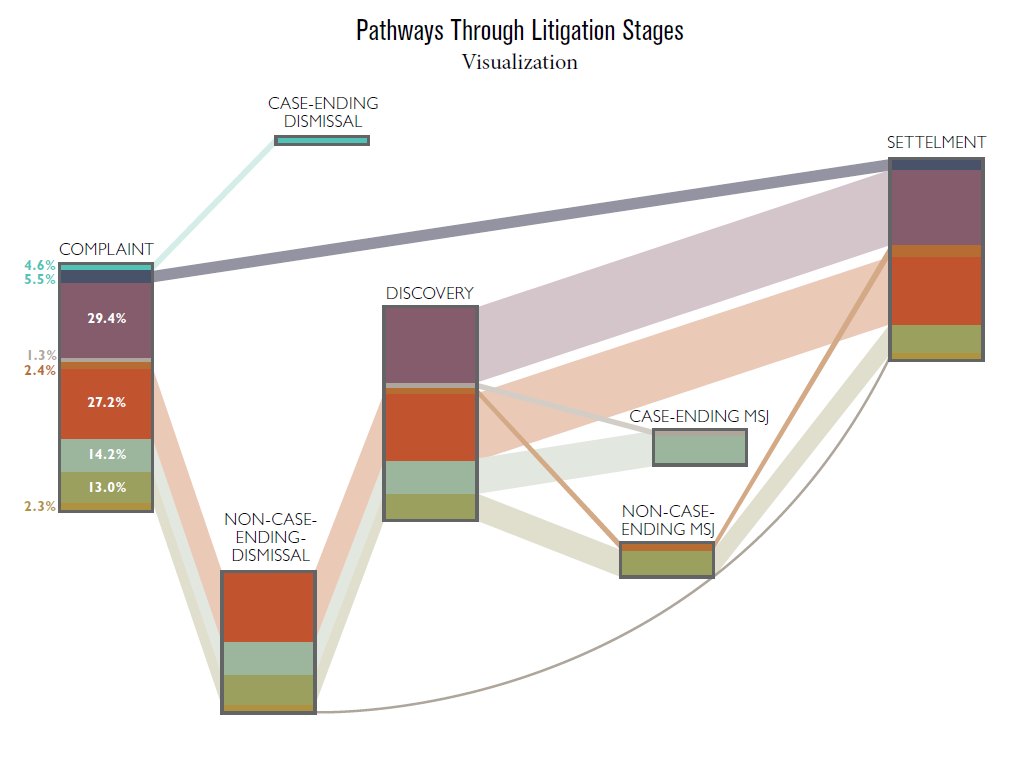
Litigators are already trying to use data analytics for jury selection, but most of this is still in a fairly nascent stage. For example, they mine social media for information about particular potential jurors that might indicate their (un)suitability for jury selection. But what if data analytics were applied to all of the questions and all of the answers and all of the outcomes of all of the juries in a particular jurisdiction? It is conceivable that certain responses to a question set developed out of that exercise could accurately predict the outcomes of jury trials in a particular kind of litigation.
It doesn’t stop there. Some companies already use data analytics to help prevent litigation by identifying particular behaviors that historically have resulted in litigation and using training and policy changes to try to mitigate those behaviors. But what if the results of standard employee surveys could be used as a predictor of certain kinds of compliance or litigation risk, to help mitigate that risk?
These kinds of developments in data analytics can, and perhaps should, be a little scary to most of us. The idea that our innocent responses to apparently unrelated questions might be used to identify any of us as a potential compliance risk or to disqualify us from a jury may make us a little uneasy. But all signs point to this kind of thing developing whether it makes us uneasy or not.
We need not only understand these developments so we can take advantage of them on behalf of our clients, but also so we can advise our clients on how to avoid any pitfalls associated with data analytics developments.
As always, feel free to contact me if you have any questions.
Further Reading
Anne Tucker And Charlotte Alexander, “Why We’re Training the Next Generation of Lawyers in Big Data,” The Conversation, Oct. 2, 2018.
Charlotte S. Alexander, Khalifeh al Jadda, Mohammad Javad Feizollahi, and Anne Tucker, Georgia State University College of Law, Legal Studies Research Paper No. 2018-13 Using Text Analytics to Predict Litigation Outcomes: A Preliminary Assessment and Law as Data: Computation, Text, And The Future Of Legal Analysis (under contract, Santa Fe Institute Press, Michael Livermore, and Daniel Rockmore, eds., 2018, forthcoming).
Anne Tucker And Charlotte Alexander, Why We’re Training the Next Generation of Lawyers in Big Data,” The Conversation, Oct. 2, 2018.
Lisa Dane, “Leveraging Social Media Analytics in Jury Selection,” The National Trial Lawyers, April 12, 2016.
Jason Tashea, “Algorithms Fall Short in Predicting Litigation Outcomes,” ABA Journal, Sept. 2018.