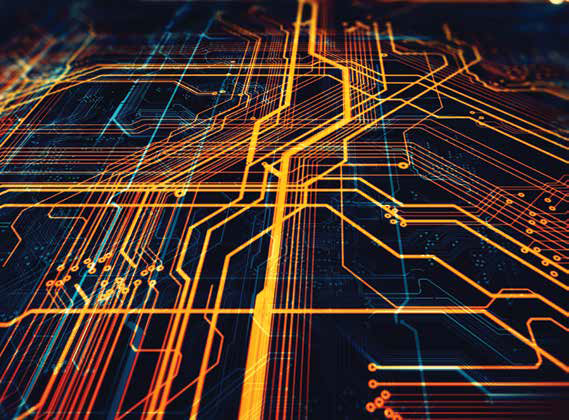
In 1950, Alan Turing proposed what has become known as “The Turing Test,” which is a test of a machine’s ability to display intelligent behavior indistinguishable from a human. Turing proposed that a test subject would separately interact with both a machine and a human through a text-based interface. If the test subject could not reliably distinguish the machine from the human respondent, the machine would be considered to have passed the test. Note that Turing’s insistence on a text-based interface was so that the test subject would not be able to identify the machine based on the quality of the machine’s speech.
If Turing were to formulate the same test today, he might not have bothered about the latter. Google introduced “Google Duplex: An AI System for Accomplishing Real World Tasks Over the Phone” a few months ago. If you listen to the demo, I think you will be astonished at how natural and human-sounding the AI is. Although Google was very clear that the product is in its very early stages and only “works” about 80 percent of the time, the internet quickly descended into a swirling debate about the authenticity, ethicality, morality, and usefulness of the product. One discussion took the position that they couldn’t imagine businesses opting to use a tool that was still so crude.
I had to laugh. I had just spent several incredibly frustrating phone calls trying to get past various robot receptionists. They kept giving me a limited and inappropriate set of options to choose from, couldn’t understand my responses, and inevitably, after my frustration level had reached a fevered pitch, finally put me in touch with a human. And the robot voices sounded exactly like what they were — socially tone-deaf machines. Talk about crude!
But this got me thinking. I had recently returned from the ACC Legal Operations conference in Chicago (which I highly recommend), and there was a very interesting presentation on the present and future of AI by Sterling Miller, the general counsel and corporate secretary at Marketo, which gave me food for thought. Here is what I believe is the problem with those call center robots and with Apple’s Siri, Amazon’s Alexa, Google’s Home Assistant and Google Duplex, and all the other current talking machines, and why I don’t think it will be a problem for much longer.
I have written in the past about the way in which you can use logic trees to build smartguides, and the robot receptionists operate on exactly the same type of architecture. In essence, if I call my phone company, there are clearly a limited number of things I am trying to do. And so the robot asks me to pick the option that best applies to me. Once I have gone down that branch, there are then just a few more options that can apply to that branch, and so on. With luck, I will be directed to the information that I’m looking for.
The trouble with most robot receptionists is that the humans who designed the logic trees didn’t, for whatever reason, do a very good job. I’m not saying there aren’t occasions when what I or some other caller is trying to do isn’t an edge case, but I often find myself frustrated by the simplest of requests not fitting into their logic tree. And if I really am exhibiting an edge case, it takes far too long to route me to a human.
This is, in my opinion, frankly inexcusable when it comes to calling a phone or cable company. The variables and consequent logic trees should be extremely simple. But if you think about the extent to which a robot telephone operator could one day serve as a combined operator, legal request portal, and interactive smart-guide to either provide simple responses to client questions or route them to the right digital or human resources, you get into a much more complicated logic tree.
But this kind of more complex logic tree is somewhat similar to the one Google is trying to build in Duplex. Duplex is currently being developed as a personal assistant that could make restaurant or other reservations in the same way a human could. But because the idea is to create an assistant that seems human, the assistant has to be able to parse/interpret a wide array of human speech formulations. Humans often ask what are in essence the exact same questions in a multitude of ways, so the logic tree that ensures it takes the correct branch can become very complex. An 80 percent effective rate is clearly not ready for market, but it is truly impressive when you consider the complexity of the task. How did Google do it? In their own words:
To obtain its high precision, we trained [Duplex’s recurrent neural network] on a corpus of anonymized phone conversation data. The network uses the output of Google’s automatic speech recognition technology, as well as features from the audio, the history of the conversation, the parameters of the conversation (e.g., the desired service for an appointment, or the current time of day) and more. We trained our understanding model separately for each task, but leveraged the shared corpus across tasks.
In other words, Google is using machine learning to create complex logic trees built upon the “experience” of the neural network in successfully communicating with humans in somewhat the same way that IBM’s Watson “taught itself ” Chess, Go, and Jeopardy. It may take five to 10 years, but I can easily imagine a future in which your law department has a robot that answers your telephone in a pleasant human sounding voice: “Hello, I’m John, the Legal Department’s AI. How can I, um, be of assistance?”