Banner artwork by Askhat Gilyakhov / Shutterstock.com
Technology has upended the practice of law. While it has created legal issues that did not exist a few years ago, it has also created opportunities to handle increasingly complex matters efficiently.
One area of development, and the focus of this piece, is the application of data science to the practice of law: legal data science. This discipline refers to the use of scientific methods, processes, algorithms, and systems to extract insights from large-scale data to further legal work. Specifically, legal work benefits from the massive increase in and ease of producing, storing, and analyzing data. Legal teams with access to data, the know-how to ask questions about them, and the technical ability to answer these questions gain a professional advantage compared to peers without data science-savvy.
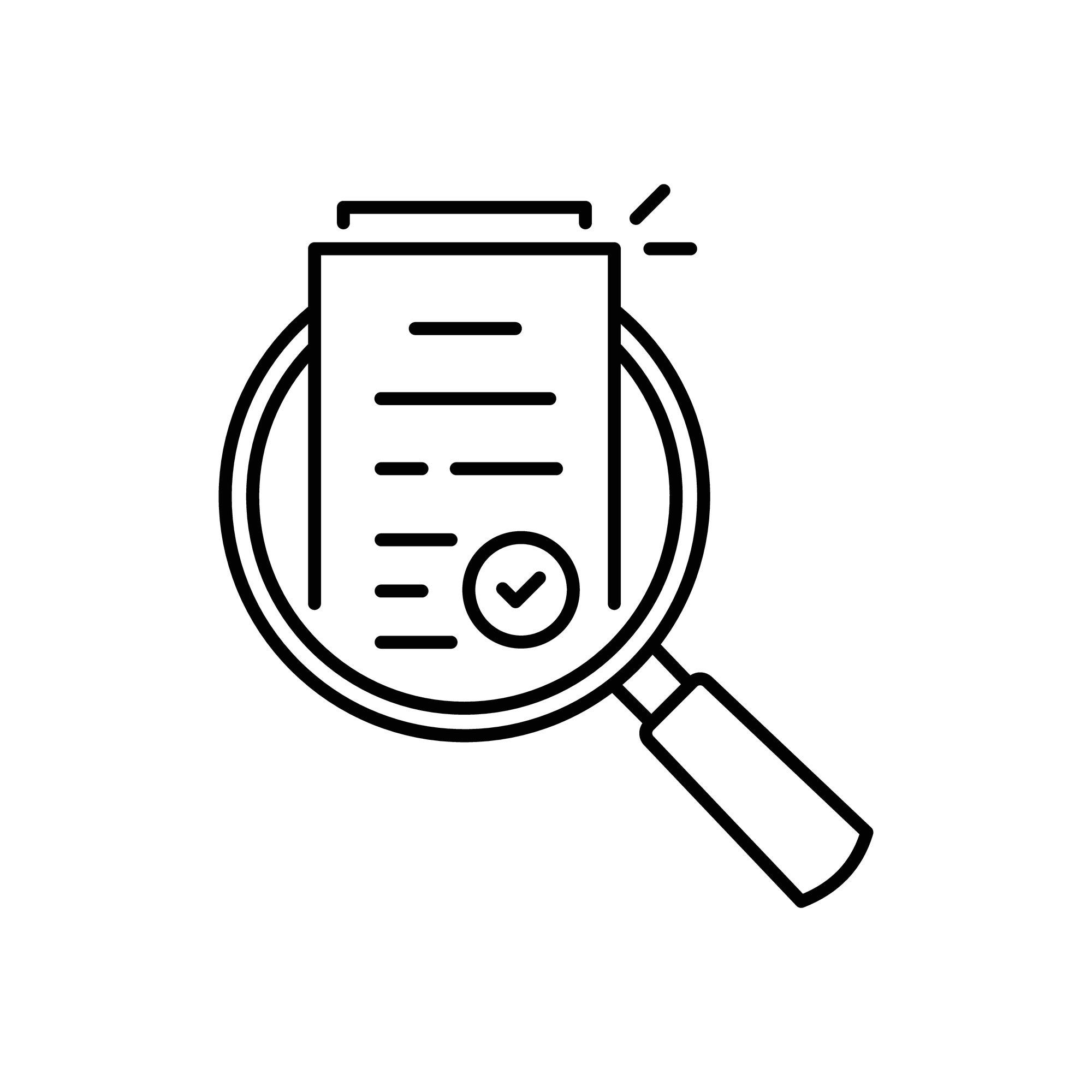
Legal practitioners have many use cases for data science. They include analyses and visualizations to support arguments in litigation; analyses to support regulatory approval for mergers and acquisitions; quantification of legal exposure; legal operations analysis, from spending to staffing; data productions in litigation and regulatory matters; and machine learning to predict litigation outcomes and detect compliance risk.
In-house legal practitioners who want to take advantage of legal data science have three options at their disposal. They can:
- Contract with external consultants,
- Work with in-house data scientists in business units whose primary responsibilities are not legal work, or
- Hire data scientists in the legal department dedicated to supporting the legal department full-time.
Each of these options has different advantages and disadvantages. Here, we offer an overview of these three options and how Uber’s legal department has combined them to meet its data science needs across global legal work that spans litigation, competition, intellectual property, investigations, employment, privacy, and compliance.
3 approaches
Option 1: External consultants
The first, most approachable option to in-house counsel familiar with working with outside counsel and experts, is to hire an external consultant. Many consulting firms offer data science services to legal departments, and law firms are increasingly offering legal data science services to interested clients.
Hiring external consultants to provide data science expertise instead of building it in-house, saves the in-house legal team the difficulty of hiring and managing a legal data science team, which is still a relatively niche discipline. In addition, this option allows in-house legal teams to benefit from the data science best practices that a consultant has learned from various clients.
Hiring external consultants to provide data science expertise instead of building it in-house, saves the in-house legal team the difficulty of hiring and managing a legal data science team, which is still a relatively niche discipline.
But this option presents several challenges. First is the speed with which a legal team can hire, onboard, and deploy an external consultant. In particular, each company has unique data infrastructure and needs. Hiring the same consultancy across multiple engagements can reduce data onboarding costs, but consultants may not be able or incentivized to make long-term investments in their tools and solutions to make repeat work faster over time.
External consultants may also be focused on specific short-term tasks rather than longer-term thought partnership with the legal department on emerging legal issues that could benefit from a data scientist’s expertise (e.g., partnering with in-house lawyers in assessing risks associated with the implementation of algorithms and machine learning in a company’s business).
These challenges could be more pronounced in larger legal teams in which different specialty practice areas retain different outside counsel firms. For example, if an outside firm does privacy work and another firm does employment work, it will be harder to identify common data science needs and build solutions that address these across specialties.
Option 2: Partnering with data scientists from existing business units
The second option, relying on business-side data scientists who sit outside the legal department but in the same organization (e.g., in a data science department), mitigates some of these challenges without having to hire, manage, and retain data scientists in the legal department. General data scientists can support legal needs, bringing their expertise with internal data to bear on legal matters while being familiar with internal tools to analyze these data as part of their regular work. The legal team benefits from already-established best practices. And they are potentially not directly responsible for the financial cost of these data scientists.
But this option also presents its own set of challenges. It requires tight coordination and prioritization with the department funding data science headcount. This is difficult when legal priorities differ from business priorities. And when legal work is prioritized, data scientists may be unfamiliar with the legal context and more incentivized to create short-term solutions to legal problems rather than taking a more holistic perspective on legal data science needs and making long-term technical investments to address them. For example, business-side data scientists may be less likely to notice the kinds of software tools and datasets whose creation would solve legal data science challenges across legal practice areas.
Option 3: Hiring data scientists directly into the legal department
A third option involves hiring in-house legal data scientists. This team is dedicated to and gains experience in solving the data science needs of the legal department, gaining a delivery speed advantage over external consultants and business-side data scientists.
Legal data scientists are familiar with internal data and tools. They can extend them to make long-term investments tailored to the recurring needs of in-house counsel, including building more complex solutions that rely on artificial intelligence. They can also serve as technical liaisons between the business and in-house counsel, communicating technical needs to the business and technical limitations to counsel. Legal data scientists can also serve as thought partners to in-house counsel, helping to identify new areas of work and set a strategic roadmap for their work to mirror and support the legal department’s goals and priorities.
The central challenge of an in-house legal data science team is the expertise it takes to hire, manage, and retain them, especially given that legal data science is a new and niche discipline. Nurturing the professional development of legal data scientists is challenging if an organization does not have sufficiently varied data science needs.
The central challenge of an in-house legal data science team is the expertise it takes to hire, manage, and retain them, especially given that legal data science is a new and niche discipline.
Finally, data scientists rely on a broader data team — that includes job families like data engineering — to provide the most value to an organization, legal, or otherwise. Being able to support these talent management needs is a tough hurdle to clear for legal departments in many organizations.
Uber's hybrid approach
Over the last few years, Uber’s legal department has met its data science needs with a combination of the three approaches outlined above to try to benefit from each approach’s advantages while mitigating its corresponding disadvantages.
At the center of our approach is a small in-house legal data science team. Their work centers on the analysis, visualization, and production of data across litigation and regulatory matters (e.g., creating trial demonstratives or producing data to comply with regulatory requests). It also includes internal use cases, like quantifying legal risks and analyzing billing data (e.g., outside counsel spending).
The team’s work has increasingly grown to include machine learning. For instance, developing machine learning models to support litigation and regulatory use cases.
In addition, the legal data science team makes long-term investments, like making self-service tools that empower paralegals and lawyers to analyze data on their own. For example, our team has written dozens of data analysis scripts that are stored in a user-friendly interface for paralegals and counsel to use by specifying inputs relevant to specific matters.
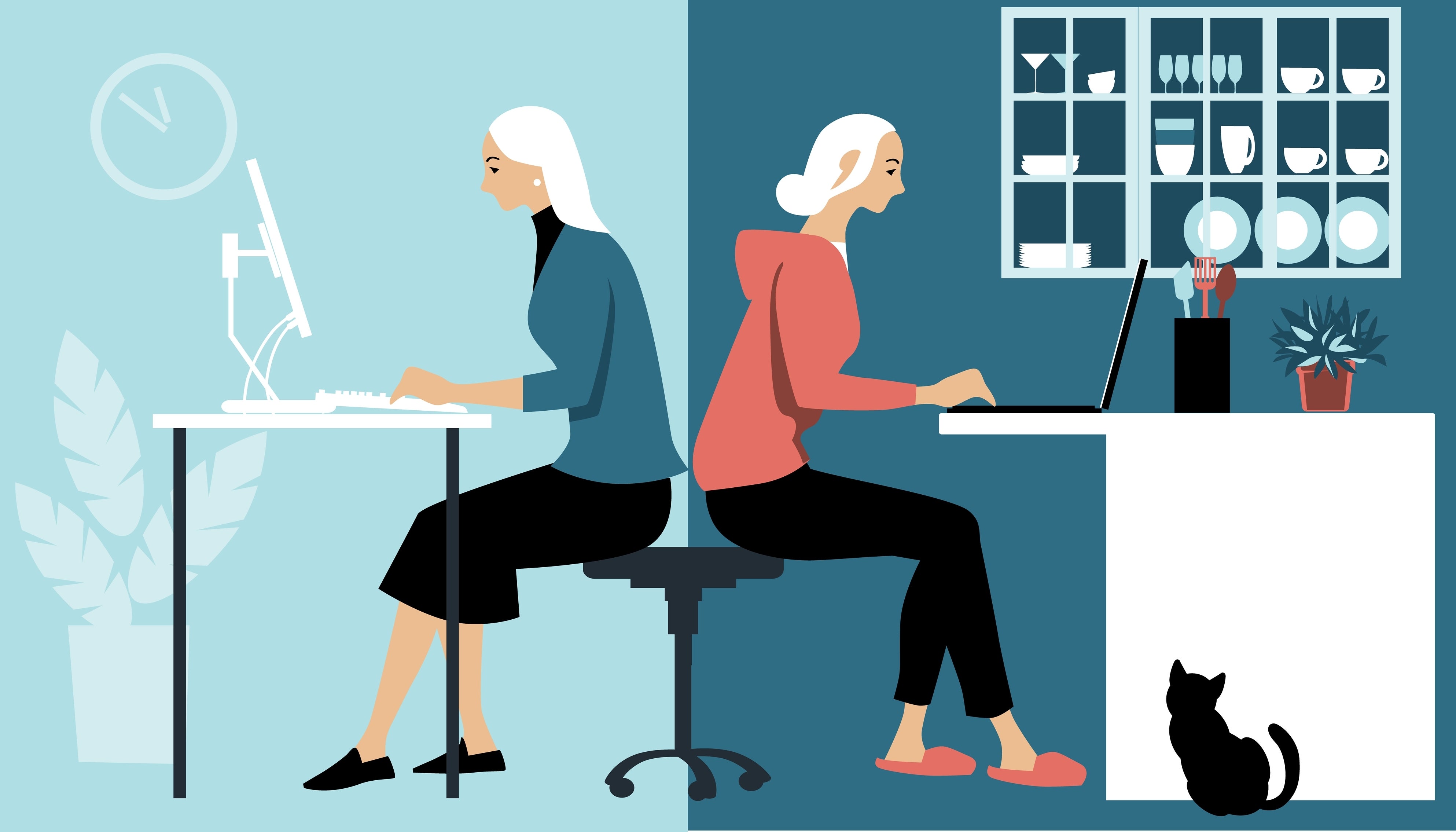
A data science manager supervises the team but is part of the litigation team. Embedding the team in the legal department makes it easy for team members to build strong relationships across the department, focus on the legal department’s priorities, and grow their command of and familiarity with legal matters across geographies. It also allows legal data scientists to serve as thought partners to in-house counsel, identifying new areas of work (e.g., partnering with lawyers to advise the business on emerging risks related to the use of algorithms and machine learning) and strategizing on more complex technical projects (e.g., identifying novel visualizations to use as trial demonstratives).
The legal department still relies on business-side data scientists, who replace or augment the work of legal data scientists for work that requires business expertise, is well-scoped and easy to deliver, and when their bandwidth allows them to take on the work. But even here, the legal data science team often serves as a technical liaison between counsel and other technical teams in the company (e.g., business-side data scientists, software engineers, product managers, etc.).
External consultants also help in-house counsel meet their data science needs. They are hired strategically for infrequent and staffing-intensive mergers and acquisitions projects and as consulting or testifying experts for complex litigation. But even here, in-house legal data scientists help these consultants navigate internal data and liaise with business-side technical teams. Finally, legal data scientists partner with external consultants to craft study methodologies and furnish the best data for their analyses.
In-house counsel also use an eDiscovery team that is separate from the legal data science team. But these two teams collaborate closely on specific projects, like facilitating and organizing the collection of specific types of communications. The eDiscovery team shares snapshots of inbound emails with legal data scientists, who process these data to update a database that can be efficiently queried across different legal matters.
Legal data science teams may not be a good fit for all legal departments. Our legal data scientists benefit from Uber’s data infrastructure and data science tooling, which may not be available at smaller, less technical organizations.
Toward a legal data science practice
Legal data science remains a relatively new discipline, still evolving in its technical approaches and as in-house and law firms implement it. And while a legal department’s specific implementation of legal data science will depend on the magnitude and complexity of its needs, in a data-rich world, no legal teams will avoid its need.
Those in-house legal practitioners who want to take advantage of legal data science sooner rather than later will have to find the best combination of approaches for their situation.
Whatever the best implementation looks like for a given legal team, we hope that a review of these three approaches and a high-level overview of Uber’s hybrid solution sparks ideas to advance toward a legal data science practice in your organization.
The opinions expressed in this essay are the authors’ and do not necessarily represent or reflect the views, opinions, or policies of Uber.
Disclaimer: The information in any resource in this website should not be construed as legal advice or as a legal opinion on specific facts, and should not be considered representing the views of its authors, its sponsors, and/or ACC. These resources are not intended as a definitive statement on the subject addressed. Rather, they are intended to serve as a tool providing practical guidance and references for the busy in-house practitioner and other readers.